National Lab Researchers Boost Chip Design Processes With Artificial Intelligence
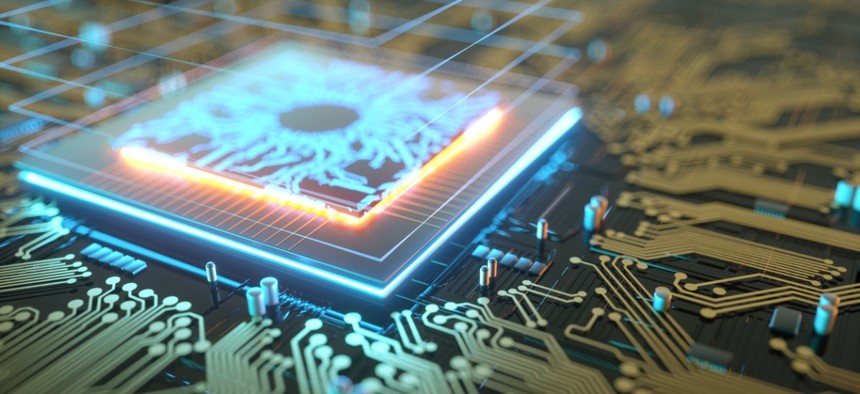
MARHARYTA MARKO/iStock.com
The experiments could hold the potential to help ease future semiconductor shortages.
Argonne National Laboratory researchers uncovered and continue to explore new ways to advance a semiconductor chips design technique using artificial intelligence.
They present several AI-based approaches to optimize atomic layer deposition, or ALD, processes in a recently published study. The method produces super-fine films of materials, like one atom thick.
It also partly underpins the making of computer chips, which are now at the center of a global supply chain shortage that’s pushed up prices of all sorts of electronics.
“The effort predates the current chip shortage issues, but we have been looking at semiconductor processing and its manufacturing challenges for a long time,” ARNL Principal Materials Scientist Angel Yanguas-Gil told Nextgov Thursday.
Yanguas-Gil has long pursued AI-aligned semiconductor innovation, including helping pioneer a cutting-edge neuromorphic computer chip modeled off insect brains. He explained chasing fundamental breakthroughs that could profoundly impact advanced manufacturing is a priority for Argonne. Yanguas-Gil’s group received funding from the Energy Department’s Technologist in Residence Program, among others, which he said, “encouraged [them] to look at the industry as-a-whole.” The lab has a strong ALD program, he noted, and their established connections with the private sector helped insiders fully grasp the existing key challenges.
“This led to an internally funded research program specifically focused on applying AI to manufacturing that supported the current research,” Yanguas-Gil said.
A press release recently published by the lab details the complexities of the fresh experiments.
ALD can be used to grow thin films for diverse applications. It occurs inside a chemical reactor, where “precursors,” or two different chemical vapors, cling to a surface and over time result in a fine film. The technique “excels at growing precise, nanoscale films on complex, 3D surfaces,” according to the lab’s release, motivating scientists to explore the making of new ALD materials for the next generations of devices.
Forming and optimizing nascent ALD processes is incredibly tedious. So Yanguas-Gil and the lab team moved to explore three optimization strategies: random, expert system and Bayesian optimization.
“We can use a cooking metaphor to explain the three models,” Yanguas-Gil explained.
The Bayesian optimization is an algorithm that—as it tries various conditions and receives feedback—learns an internal model that helps it understand precisely what are the most promising conditions to try next, he noted. In cooking terms, that algorithm has absolutely no prior information about what “cooking” means—it simply starts with a collection of ingredients and oven setting.
“It is really good at quickly figuring out the proportions of ingredients that lead to a good recipe based on the feedback it receives,” the scientist explained.
In contrast, the expert system builds on earlier types of AI that attempt to codify some expert information about trends that it should expect during the optimization process. To find the optimum condition, the system uses those expectations or rules together with the feedback it receives after trying a new condition. Considering the cooking analogy, “it is as if we tell the algorithm that it should focus on the proportion of the main ingredients first because you can always rectify the spices once the dish is cooked,” Yanguas-Gil said.
And the random system is the baseline case, he added. Through it, they’re essentially “just picking up conditions at random, hoping that one of them is a good one.” Eventually, the system will land on a point that may be close enough to the target—“but you have to be willing to taste really gross dishes in the process,” according to Yanguas-Gil.
“The random system helps us get a sense of how hard—and how gross—it is to get to a really good recipe,” he noted, “but it is not a method that is really intended for the user.”
Lab researchers evaluated the three optimization strategies, aiming to find conditions that led to “high and stable film growth in the shortest time,” among other aims. The work involved optimization algorithms, a simulated system and more. Researchers also established a close-loop system, through which a simulation completes an experiment, feeds results to an AI tool. Then, the AI tool interprets those, and recommends the next experiment—all without any input from humans.
The AI-based approaches determined optimal timing elements for different simulated ALD processes. The study is “among the first to show that thin-film optimization in real-time is possible using AI,” the lab’s release confirmed.
To Yanguas-Gil, coupling in-situ techniques—or examining events where they happen—with machine-learning algorithms to drive optimization “seems like a no-brainer.” But he and his team weren’t able to identify any meaningful examples applied to ALD across scientific literature that predated this work.
“I think that part of the reason is that you need a team with a diverse range of expertise working together: experts in the technique, both in terms of fundamentals, in-situ characterization, and manufacturing, experts in machine learning, in modeling and simulation, experts in instrumentation,” Yanguas-Gil said. “We happened to have that at Argonne.”
The research provides a means to accelerate integrating new processes into manufacturing. It also introduces the chance that such approaches could help America’s makers save time and money amid chip development.
“The actual impact is going to depend on tool manufacturers or specialty fabs picking up these ideas and applying them to their own problem,” the researcher noted. “Our mission is to put the technology out there and help industry in any way we can to get new capacity online as fast as possible.”
Going forward, he and his team have multiple concepts for how they can “push this research further.” And they are also thinking about technology transfer. In that light, Yanguas-Gil added that an advantage to the fresh approach is that it works with off-the-shelf characterization tools.
“We are having conversations with industry—companies interested in understanding how this research can help them optimize their processes,” he said.