DOD-Backed Effort Will Use Supercomputing to Predict Therapies for Future COVID-19 Variants
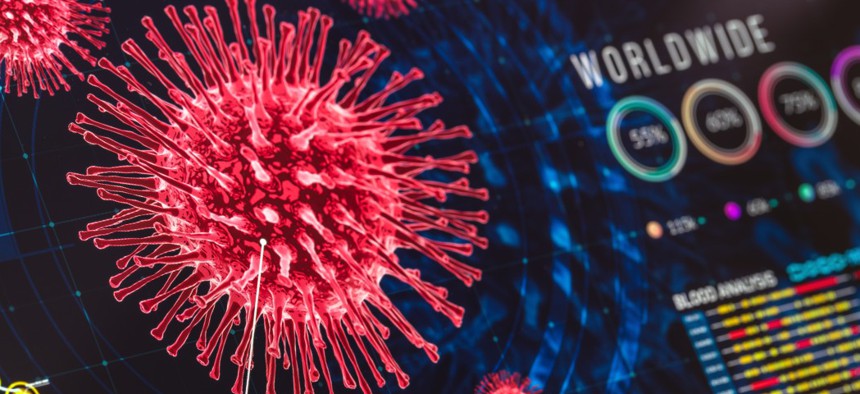
sankai/Getty Images
The aim is to build predictive models that might significantly reduce the time it takes to respond to yet-to-be-seen COVID-19 variants, once they surface.
With plans to apply synthetic biology and machine learning to speed up the discovery of therapeutic antibodies to fight against potential COVID-19 variants, Lawrence Livermore National Laboratory and A-Alpha Bio researchers joined forces this week.
A Defense Department subcontract valued at $1.1 million will finance this fresh effort.
“Developing tools to rapidly respond to emerging coronavirus variants, the focus of this work, is enormously important and high-impact,” A-Alpha Bio CEO and Co-Founder David Younger told Nextgov on Wednesday. “However, COVID-19 is just the starting point—if we are successful here, we can apply the same approach to other rapidly evolving infectious diseases and biothreats, which would be an enormous leap forward for public health and biosecurity.”
Through this effort, the company will essentially measure millions of interactions involving proteins between ML-generated antibody libraries and panels of coronavirus variants. The national laboratory will use that to refine its own ML models for predicting antibody sequences that could work well to treat variants of concern and potential future variants that may surface.
LLNL is one of multiple government labs to deploy data-driven and predictive simulation capabilities in response to the pandemic—and this new contract will continue to push such work.
The partners are “essentially preparing computational models to predict therapeutics for any potential emerging mutation,” Younger noted, diving deeper into this joint engagement. He offered an analogy to help make sense of the pursuit.
“What we're essentially proposing to do here is start from an antibody that binds to an existing Coronavirus strain like the Wuhan Coronavirus, for example. And we can think of that ‘binding’ as a key fitting into a lock,” he explained. “Imagine now that there's been a mutation. The lock, which, in this analogy, represents the Coronavirus, has mutated. It has changed. It has become the delta variant, for example.”
It’s still really similar to the previous variant—so not a whole new lock, just one with an internal mechanism that has been ground down to some degree, Younger added. The “key,” which represents the antibody that fits the “lock” of the Wuhan variant continues to work, but not as as well.
“What we want to do as antibody engineers is design a new key, one that fits just right. The problem is that this takes a considerable amount of time. A new variant emerges and the challenge is to try and find the right key. It takes months, under even ideal conditions. What we are doing with LLNL is testing thousands or tens of thousands of keys against many different locks simultaneously. That allows us to map out the landscape of possibilities—the many potential relationships between locks and keys before a new Coronavirus variant emerges,” Younger noted. “And if we repeat this process multiple times we can use the incredible volume of data we collect in the process to build new machine learning models.”
It’s impossible to predict what the next COVID-19 variant will actually look like, but the researchers hope their approach will significantly reduce the time it takes to create new treatments down the line when they do inevitably pop up.
“Here’s what might be possible once we have a fully trained predictive model—the ultimate goal of this kind of project. Imagine a new sequence, a new variant, is identified somewhere in the world. The hope is that we would input it into the model and within a day or so the model would return results: antibodies that it predicts are going to be therapeutically effective,” Younger confirmed.
This collaboration will unfold at the California-based national lab, as well as the company’s headquarters in Seattle.
“The work we’re doing with LLNL assumes that coronaviruses are going to be with us for a long time,” the CEO said.
NEXT STORY: Dairy Robot Checks Cheese Quality in Seconds