How VA Implements Predictive Analytics to Help Prevent Veteran Suicides
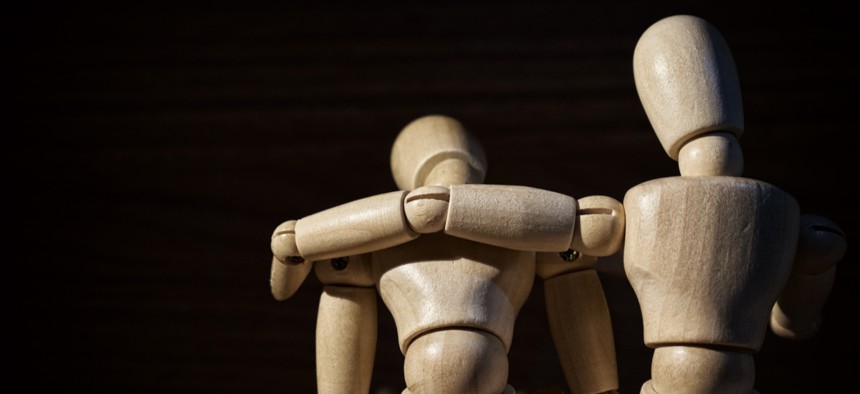
xinlan/Shutterstock.com
Since it was stood up in 2017, the REACH VET program has helped health care providers better identify individuals facing higher risks.
The Veterans Affairs Department has put technology to use in recent years to promote suicide prevention efforts and curb the higher rates of self-harm that veterans face compared to their civilian counterparts.
In recognition of September as suicide prevention month, an agency insider detailed how VA is leveraging predictive analytics and other evolving technologies through its REACH VET program to help health care providers identify those most at risk already in the department’s system before it’s too late.
“Ultimately the provider taking care of the veteran has to have the information that they need,” Deputy Director for Innovation and Program Development Aaron Eagan told Nextgov. “As health care gets more complex, it gets incredibly difficult for providers to sort through all the information available and get all the pieces they need that day to make all the decisions they need to make—and that’s where we really continue to do more.”
To agency officials, the growing public health crisis and its impact on the veteran population are increasingly dire. On Monday, the agency released the 2019 National Veteran Suicide Prevention Annual Report, which suggests that of the 45,390 American adults who died from suicide in 2017, 6,139 were U.S. veterans. Results also indicate that more than 6,000 veterans died by suicide in each year between 2008 to 2017 and that, in the most recent year, the suicide rate for veterans was 1.5 times the rate for nonveteran adults.
But Eagan, a nurse with an advanced degree in public health and over a decade of VA service, said it was back in the summer of 2016 that the agency expressly prioritized suicide prevention as an agencywide priority and tapped him to build and implement REACH VET—or Recovery Engagement and Coordination for Health-Veterans Enhanced Treatment.
“REACH VET is really a story about clinical practice in our system,” he said.
The Veterans Health Administration has about 450 suicide prevention coordinators and thousands of mental health providers and primary health care providers who work directly with veterans each day. Using a predictive model that’s continuously updated, REACH VET analyzes data within veterans’ health records to identify patients “at a statistically elevated risk for suicide, hospitalization, illness or other adverse outcomes.” If an individual is flagged in the system, their health care provider is notified and will then decide to offer pre-emptive care and support, in some cases even before a veteran feels suicidal urges.
“They know the veteran, they look at opportunities perhaps to enhance their care, and they talk to the veteran about what their risks are, what their needs are and what can be done to better meet those needs,” Eagan said. “That’s really intentional on our part to build it that way because we think predictive analytics, machine learning, whatever it is, technology has to ultimately touch people—and in our people, it has to improve the lives of our clinicians and our veterans who are in our system.”
Eagan said the model behind the program is the result of “probably eight years of work” and collaboration between researchers from the VA, National Institute of Mental Health and Defense Department. The model looks at a variety of risk factors, such as homelessness for example, as well as the relationships between those factors and the totality of them all to create a risk score, which then indicates to providers whether the veteran has entered a population of increased risk and therefore needs specific attention or resources.
But Eagan also made it a point to note that the information remains private within VA’s secure systems and also does not implement machine learning on top of its predictive system.
“The model itself is being updated because models shouldn’t be static, but I will say that one of the challenges in applying predictive analytics and providing that information to clinicians, is it’s really important that clinicians understand why the veteran is being identified,” he said. “But that is really hard to do with a true machine-learning approach because we don’t really know why the machine is identifying somebody.”
At this point, it isn’t something that veterans necessarily opt in or out of, but it’s become part of the agency’s routine care offerings. And Eagan said, for insiders within the VA, it’s been incredibly rewarding to see the emerging technology’s impact. In the first year that REACH VET was implemented, the program enabled health care providers to identify and proactively support 30,000 at-risk veterans. The following year, that number jumped to 65,000 unique high-risk veterans in need.
“That’s a lot of people,” he said. “And so that’s actually one of the most interesting kinds of findings, is that veterans have been unbelievably receptive to knowing that their providers are taking every opportunity to make sure that their needs are being met and actually reach out to them proactively to talk to them about their care and sort of what their priorities are.”
Now that the program has been fully implemented since it was initially stood up in 2017, Eagan and his team are working on a full report of its results and impact to be finalized and published as a piece of peer-reviewed literature in the near future. The agency also has big plans to build the technology behind the REACH VET program into other systems that health care providers can then use to continue to better inform their clinical decision-making.
In closing, he made a point to note that the VA’s mission to serve those who served will continue to govern every new installation or rollout.
“Sometimes we get caught up in technology for technology because it’s cool and we are very much used to it in our lives now,” he said. “But in medicine and in clinical care, it’s always about how it’s touching the veteran and we have to think of that upfront.”