New Tool Fights Fake News by Exposing the Websites That Create It
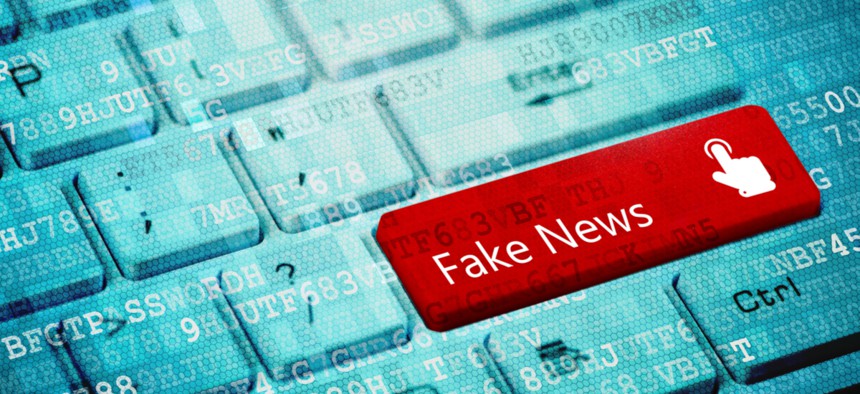
BigNazik/Shutterstock.com
MIT and Qatari researchers say it’s easier to expose a liar than debunk each of his lies
Many sites that generate fake news — disinformation masquerading as truth — share characteristics that distinguish them from journalistic outlets, according to researchers from MIT and the Qatar Computing Research Institute, who incorporated several of those characteristics into a dataset and then trained an algorithm to identify them. Their work could help fight a growing problem that many experts in government forecast will only get worse.
Facebook, Twitter, and other social media outlets are building teams of fact checkers and supporting nonprofit organizations like First Draft to spot disinformation. But fact checking and verification takes a lot more time than pushing out disinformation. Also, fake news doesn’t always match an expected pattern. Russia disinfo watchers have long observed that a key Kremlin tactic is to validate conspiratorial ideas on both sides of a given political debate (with the exception of gun control, to which they catered exclusively to pro-gun perspectives.)
That’s why fighting disinfo piece-by-piece is like bailing a boat that’s filling up faster than buckets can handle. What’s worse, research has shown that news readers of all political persuasions become defensive and resistant to the idea that news they’ve accepted is fake, especially if the act of accepting—and then sharing—that news item furthered their standing within a selected social group.
All of this is why fake news spreads faster than accurately sourced articles, including ones that debunk conspiracy theories and disinformation.
“Automatic fact-checking lags behind in terms of accuracy, and it is generally not trusted by human users. In fact, even when done by reputable fact-checking organizations, debunking does little to convince those who already believe in false information,” the researchers write.
Their study, “Predicting Factuality of Reporting and Bias of News Media Sources,” forthcoming in the Proceedings of the 2018 Conference on Empirical Methods in Natural Language, reveals key features of false news web sites that might be less visible to human fact checkers but can tab a bad news source.
Among the features: specific patterns of so-called “function words” that give a more spoken feel to a news article, as opposed to the far more common “content words.” Mainstream news editors clamp down fast and hard on too many function words, but fake news sites may not be edited at all. The number and pattern of words that seem to express some sort of sentiment is another easy giveaway, as is the amount of user engagement and shares; linguistic indicators of bias around specific topics, (or bias generally), also work.
If a news site pumps out a lot of articles with a variety and high degree of these linguistic characteristics, you can safely infer that they’re more likely to be publishing “news” that, well, isn’t.
The researchers found that their algorithm, called the Support Vector Machine, could correctly deduce a high, low, or medium level of “factuality” about 65 percent of the time. It could predict right- or left-leaning bias about 70 percent of the time. While not perfect, it’s a big improvement over a raw guess (50 percent). The authors caution that the algorithm would work best with human fact checkers.
The next step, they write, is “characterizing the factuality of reporting for media in other languages. Finally, we want to go beyond left vs. right bias that is typical of the Western world and to model other kinds of biases that are more relevant for other regions, e.g., islamist vs. secular is one such example for the Muslim World.”