How the CIA is Working to Ethically Deploy Artificial Intelligence
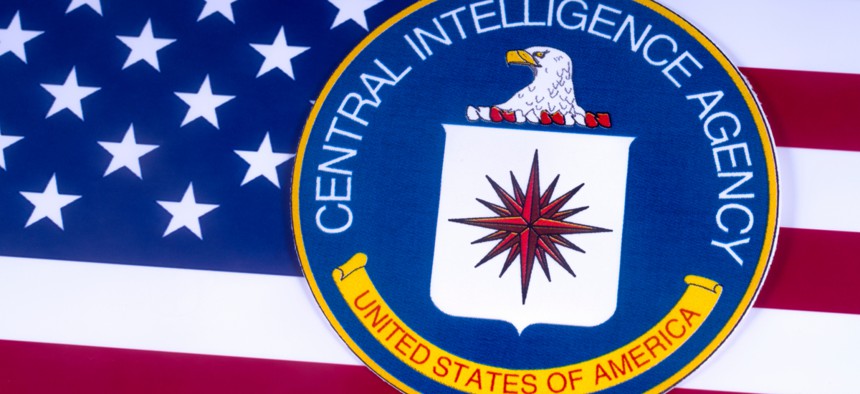
chrisdorney/Shutterstock
As the agency utilizes new technology, insiders are thinking critically about issues around privacy and bias.
As the Central Intelligence Agency harnesses machine learning and artificial intelligence to better meet its mission, insiders are aggressively addressing issues around bias and ethics intrinsic to the emerging tech.
“We at the agency have over 100 AI initiatives that we are working on and that’s going to continue to be the case,” Benjamin Huebner, the CIA’s privacy and civil liberties officer said Friday at an event hosted by the Brookings Institution in Washington. “That’s a big complicated issue that we are very much thinking about all the time.”
Huebner said collaborating with the intelligence agency’s data scientists is one of his favorite parts of his job. His privacy team works directly with their tech-facing colleagues on projects around statistics, coding, and graphical representations.
“And some of [the work] is utilizing new analytics that we have, particularly for large data sets, to look at information in ways that we weren’t able to do before and to use improvements in machine learning to see insights that as humans, just from a capacity standpoint, we can’t see,” he said.
The PCLO equated today’s landscape and the budding technology to the 1970s and 80s when federal workers began using computers for agency work and in the “early days” when the Federal Bureau of Investigation began using automobiles.
“I don’t actually like when people look at AI as something that’s so functionally different,” he said. “This is one of the tools that’s going to be used in a lot of different places.”
But the boom around the innovative technology does not come without consequences, particularly around bias and explainability, which Huebner said the agency is addressing head on.
“One of the interesting things about machine learning, which is an aspect of our division of intelligence, is [experts] found in many cases the analytics that have the most accurate results, also have the least explainability—the least ability to explain how the algorithm actually got to the answer it did,” he said. “The algorithm that’s pushing that data out is a black box and that’s a problem if you are the CIA.”
The agency cannot just be accurate, it’s also got to be able to demonstrate how it got to the end result. So if an analytic isn’t explainable, it’s not “decision-ready.”
Huebner also said his team is working directly with the CIA’s data scientists to mitigate bias in AI that the agency is implementing.
“When you are thinking about things like bias, the training data or how you train that machine learning analytic, that’s where some of the bias really can seep in, so you really want to know about that,” he said.
Sometimes that data could be useful to train an algorithm, but it may also include private information without foreign intelligence relevance. Huebner and his team are tasked with working out how to balance using the appropriate data to train machines, while upholding strict privacy measures.
He said, right now, his office is looking to develop a practical framework for insiders to use around new projects that forces them to initially ask themselves thoughtful questions around privacy, explainability and bias, ahead of delivering new analytics for mission use.
“It’s great that people are using [the tech] in the commercial space, but we are not pushing you to a better brand of coffee here—we need more accuracy and we need to know how you got there,” he said.