Avoid These 5 Common Data Pitfalls to Maximize the Impact of an Analytics Program
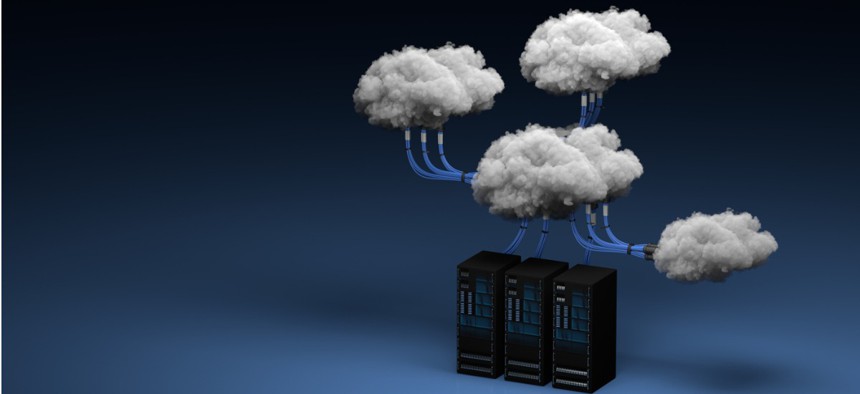
Just_Super/iStock.com
Leveraging data is incredibly hard.
Operating in an analytics-driven manner is a priority for agencies across the U.S. public sector. In part, this change is due to mandates like the Federal Data Strategy but also a desire to replicate the private sector data-first approach.
Unfortunately, leveraging data is incredibly hard. Time and again, I’ve seen groups and individuals tasked with delivering digital transformations tripped up by stalled projects, never-ending timelines and blown budgets on bad tech decisions.
Identifying these challenges and planning how to address them before embarking on a new analytics project can dramatically increase the chance of success.
Here are the five most common pitfalls that I have seen IT leaders fall prey to during digital transformations.
Pitfall 1: Not Moving Data to the Cloud
If your agency isn’t planning to migrate to the cloud, you will be subject to rising operations and maintenance costs for infrastructure. At this point, security concerns have been largely mitigated. The cloud is more elastic and cost-effective than your in-house solution, more straightforward to maintain, scalable and more likely to be fault-tolerant. With the explosion of secure cloud environments dedicated to the U.S. government, there is little reason not to make a move.
Pitfall 2: Believing Data Lakes Will Solve Data Volume Problems
Many agencies assume if they load all their data into a data lake—a centralized repository—they’ll be able to correlate all their data sets. The reality is that this action typically results in data swamps, not data lakes.
It’s a problem of garbage in, garbage out. Let’s say a quartermaster needs to account for troops working at two different duty stations. If the two record sets are merged, soldiers would be over-counted by the number of duplicates. The net result is your analytics will be garbage, and your machine learning models will fail. Agencies need to clean their data lakes with a data curation system that will solve these problems before trusting downstream analytics.
Pitfall 3: Not Solving Your Dirty Data Problem
You’ve hired data scientists, so you think you’ve got big data analytics covered. However, it’s crucial to look at how they are spending their time. Unfortunately, most of their time (typically more than 80%) is spent cleaning data and integrating it with other sources. This is not time well-spent and eventually, these people will leave; which leads to the next pitfall.
Pitfall 4: Not Planning for AI and Machine Learning to Be Disruptive
The U.S. public sector has been slow to move to AI/ML compared to many U.S. commercial companies but make no mistake: Change is coming. AI will displace some of your workers and has the potential to upend how you handle your operations.
Begin now to transition tasks that may move to AI/ML. Find creative ways in the pay band structures to hire AI/ML experts and expect to pay up for them. If necessary, contract out such tasks.
Pitfall #5: Not Willing to Combine Data Sets with Outside Agency Datasets
Agencies that feel they cannot connect their datasets with public data or other agencies’ datasets miss valuable information. There is so much emerging technology creating exabytes of usable data every day that can be useful for aiding the warfighter or achieving mission objectives. Agencies must be willing to seek out data enrichment opportunities and incorporate the resulting data into their environments.
Michael Stonebraker is co-founder of Tamr and recipient of the 2014 A.M. Turing Award.
NEXT STORY: AI Teaches a Robot How to Learn to Walk