NIST issues guidance on a mathematical approach to data privacy
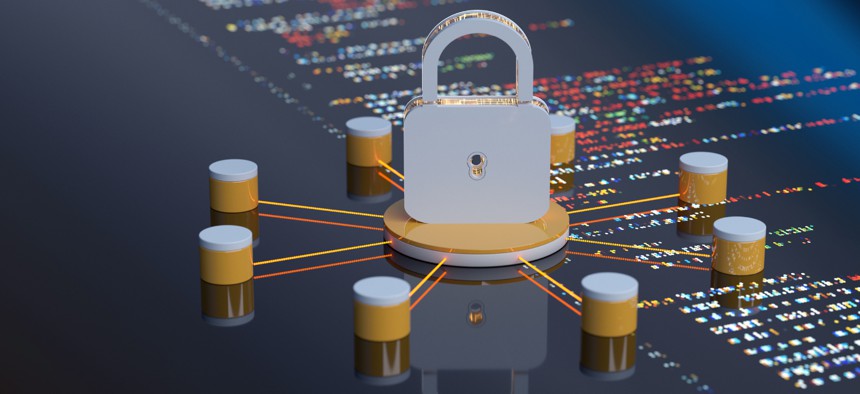
Olemedia/Getty Images
The draft document provides a system for adopting a differential privacy framework, and the agency is currently seeking feedback to ensure quality.
The National Institute of Standards and Technology launched new draft guidance earlier this week focused on clarifying how organizations can adopt differential privacy — a mathematical algorithm broadly used to quantify how much privacy risk is posed to individuals from a given dataset — as part of their security infrastructure.
As NIST’s guidance notes, differential privacy can be leveraged as a scheme to evaluate an organization’s digital privacy posture through a framework that identifies existing factors to potential breaches in data security.
The guidance is a result of President Joe Biden’s October executive order on artificial intelligence that tasked agencies, including NIST, with certain research obligations.
The goal of the NIST guidance is to help organizations strike a balance “between privacy and accuracy,” according to an agency press release. “Applying differential privacy allows the data to be publicly released without revealing the individuals within the dataset.”
A centerpiece of NIST’s guidance organizes the differential privacy framework as a pyramid: at its foundation is an organization's data collection exposure, accounting for cybersecurity protocols like access control, threat and trust models, and data collection practices.
The middle of the pyramid focuses on algorithms and correctness as other factors that can undermine data privacy efforts. Finally, the top part of the pyramid culminates in the figure epsilon, a mathematical symbol that represents the quantified strength of an organization’s privacy efforts.
Using a pyramid to represent the framework emphasizes differential privacy’s checks-and-balances approach to data security: in order to reach a strong epsilon — or privacy value — an organization must ensure the provisions at the bottom portions of the pyramid are met.
“You can use differential privacy to publish analyses of data and trends without being able to identify any individuals within the dataset,” said Naomi Lefkovitz, the manager of NIST’s Privacy Engineering Program and one of the guidance’s editors. “But differential privacy technology is still maturing, and there are risks you should be aware of. We want this publication to help organizations evaluate differential privacy products and get a better sense of whether their creators’ claims are accurate.”
The Biden administration has initiated more action in developing and testing privacy enhancing technologies, or PETs, amid the continued onslaught of escalating cyberattacks and the advancement of generative artificial intelligence systems that rely on quality datasets to make accurate, responsible decisions.
NIST has been working over the past several years to further PET development, such as through a 2022 prize challenge established between the U.S. and U.K. to develop successful PETs.
“We learned in our recent U.S.-U.K. PETs Prize Challenges that differential privacy is the best method we know of for providing robust privacy protection against attacks after the model is trained,” Lefkovitz said. “It won’t prevent all types of attacks, but it can add a layer of defense.”
NIST authors note that while helpful, differential privacy tools and techniques have yet to break into a commercial marketplace. The agency is hoping for comments about the draft guidance’s scope and topics — due Jan. 25 — to ensure the final copy is accurate.
NEXT STORY: The 2024 defense policy bill has a lot of cyber