How hackers can 'poison' AI
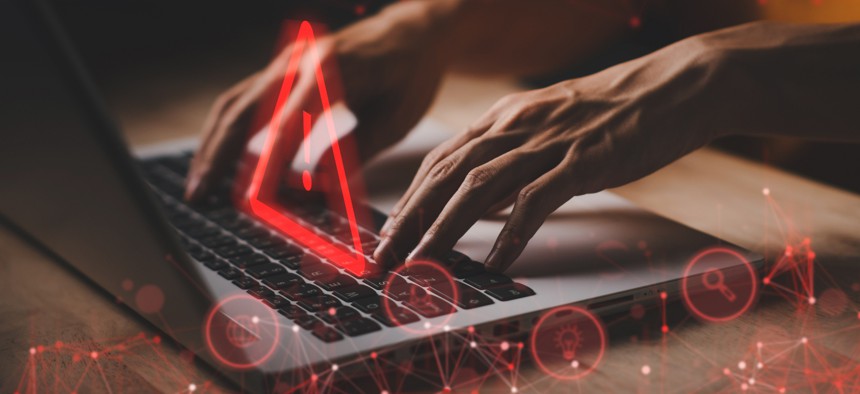
PUGUN SJ/Getty Images
A new paper from NIST offers a standard taxonomy of cyber attacks dedicated to contaminating the data AI models use to learn.
The National Institute of Standards and Technology is raising awareness of adversarial tactics that can corrupt artificial intelligence softwares. These attacks hinge on contaminating the datasets used to train AI and machine learning algorithms.
In a new paper on adversarial machine learning, NIST researchers discuss the emerging security challenges facing AI systems that depend on data training to produce accurate outputs. This dependency has allowed for the malicious manipulation of some of that training data.
The report defines the parameters and characteristics of digital attacks targeting AI/ML softwares and datasets, while also providing methods of mitigation for developers following an attack.
“We are providing an overview of attack techniques and methodologies that consider all types of AI systems,” NIST computer scientist and co-author of the paper Apostol Vassilev said in a press release. “We also describe current mitigation strategies reported in the literature, but these available defenses currently lack robust assurances that they fully mitigate the risks. We are encouraging the community to come up with better defenses.”
The four specific types of attacks the report identifies are evasion, poisoning, privacy and abuse.
In evasion attacks, adversaries attempt to alter inputs the AI system receives after its deployment to change how it responds. NIST notes one such example might be altering a stop sign so that a self-driving car reads it as a speed limit sign.
Poisoning attacks occur earlier in the AI development lifecycle, where attackers taint training data by introducing corrupted information.
Privacy attacks refer to attempts that happen during deployment, where the attacker works to learn sensitive information inherent to the AI model or its training data, or they attempt to reverse-engineer the model by inputting questions that target perceived weaknesses.
“The more often a piece of information appears in a dataset, the more likely a model is to leak it in response to random or specifically designed queries or prompts,” the report reads.
Abuse attacks further capitalize on an AI systems’ privacy by poisoning the online sources — like a website or document — that the algorithm uses to learn.
In addition to identifying distinct types of attacks, the report distinguishes different types of knowledge threat actors may possess in three categories: white-box, black-box and gray-box attacks.
White-box attacks assume the attacker has a very strong to full operational knowledge of how an AI system works and functions as zero-day scenarios.
Black-box attacks refer to an attacker with little to no knowledge of the AI system they are attempting to tamper with, while gray-box attacks can fall somewhere between the black and white spectrum.
Notably outside the scope of the report are recommendations based on an organization’s risk tolerance or the varying levels of risk acceptable within different entities. Researchers write that this is “highly contextual” to any given organization and that as such, the report should be used as an approach for assessing and mitigating the security and trustworthiness of AI systems.