Using AI, ML Will Help the Government Tackle Climate Change, Experts Say
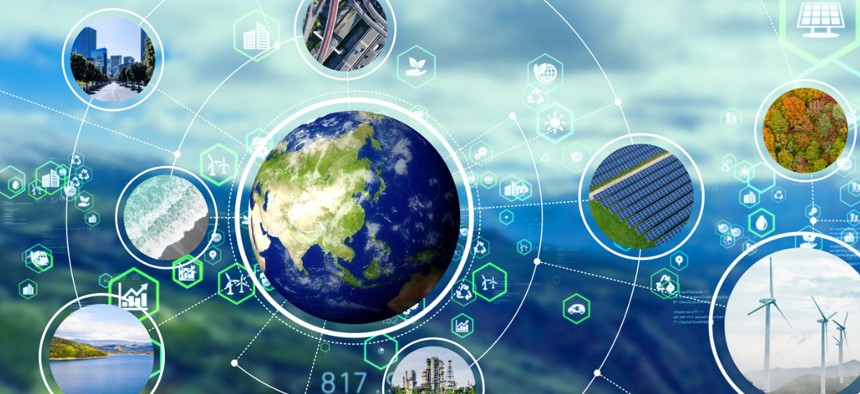
metamorworks/istockphoto
Artificial intelligence can help scientists better forecast the impacts of climate change across numerous arenas.
The frequency and magnitude of natural disasters such as hurricanes, wildfires and floods has been growing for a number of years.
Panelists for the Advanced Technology Academic Research Center’s Jan. 26 webinar “Leveraging Predictive Analytics to Address Climate Change Issues” discussed how the use of artificial intelligence and machine learning can provide both short- and long-term guidance for decisionmakers considering how to ameliorate impacts.
According to the National Centers for Environmental Information, which is part of the National Oceanic and Atmospheric Administration, in 2021 there were 20 weather and climate disaster events that incurred losses of more than $1 billion each. From 1980 to 2021, the annual average was 7.4 events (adjusted for inflation), but from 2017 to 2021, the most recent five years, the average number of events was 17.2 (adjusted for inflation).
In short, the problem is getting worse, and faster.
“One thing has changed over the past 20 years,” said Ed Kearns, Chief Data Officer for First Street Foundation, a nonprofit organization that has been assessing hyperlocal flood risks across the United States. “The change in the conversation is from ‘Is climate change happening? How do we know?’ It’s now moving to ‘How are we going to deal with it?’”
Chakib Chraibi, Chief Scientist, National Technical Information Service, said the Paris Climate Accords may have a target of limiting the earth’s warming to under 2 degrees Celsius by 2050, but current estimates are that temperatures will pass that target by 2030, which does not leave much time for mitigation.
“One factor that affects climate change is our energy use,” Chraibi said. “Energy is a key source of economic growth [and] the consumption of energy has been progressing exponentially.”
As an example, he said, “Scientists [figured] out in August 2021 that we’ve consumed 100% of the world’s renewable resources … We’re now living in a deficit.”
Chraibi said modeling climate change and its effects depends on massive amounts of data, but it has to be done at a granular level, not at a regional or national level.
Combining environmental data with economic and socioeconomic data can help identify specific communities most at risk from natural disasters, Kearns said. “At the heart of it, those data policies [that require the government to share its data] give us a fighting chance to understand what we need in order to take action, both as a nation and as local communities.” He said his organization is working on very detailed flooding risks, “house by house, business by business,” which can then be aggregated for broader policymaking decisions.
“I believe as a scientist that AI is the game changer,” Chraibi said. “We don’t follow explicit rules [in our models], we try to build a system that infers rules … Some studies have shown that the use of AI can achieve a 5-10% reduction” in CO2 emissions. “In energy, a lot of studies are involving AI because it allows you to use data for better forecasting.”
Kearns said he envisions using machine learning and AI to fill data gaps in order to link the connection between CO2 and precipitation, for instance. Then “we [try] to tie it to dollars and cents,” he said, because politicians and policymakers respond better to a measurement they understand.
“One of the best things I’ve seen over the past two years is the shift in thinking, particularly at the federal government level,” Kearns said. “At the federal policy level climate change has been viewed as kind of a science project, [a] NOAA, NASA, [U.S. Geological Survey] problem, but this administration is leading with Treasury … They need [climate information] translated into things like jobs, financial regulations, and that’s beginning to happen now.”
Chraibi said the use of AI has been rather limited until now, focusing most often on some single dimension of climate risk. “We need models that can learn from diverse streams and translate [their findings] … Machine learning models are heuristic models,” providing approximate answers with levels of uncertainty. “This is something we have to learn to work with, because that’s part of the model. What can we do to diminish [or] minimize the uncertainty?”
Kearns agreed. “One of the holy grails in science is how to convey uncertainty … If I get 95% certainty in my analysis, as a scientist I’m betting the house on that, [but] if I say that to a congressman or a reporter, they say, ‘So you’re not sure.’ We’re using the word ‘uncertainty’ but the meaning is getting lost. That’s why bringing it to dollars and cents or other” meaningful measures is important, to convert into something the broader public can understand.