National Archives Wants to Use AI to Improve ‘Unsophisticated Search’ and Create ‘Self-Describing Records’
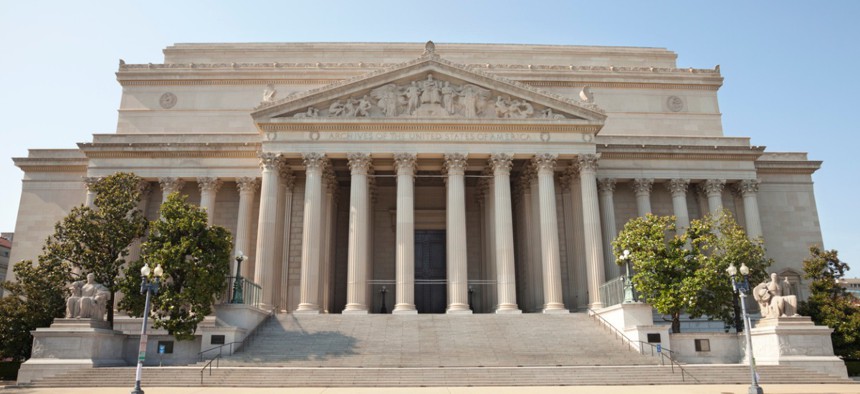
Willard/istockphoto
The National Archives and Records Administration wants to automate its records management processes to limit manual metadata tagging while improving the search function.
The National Archives and Records Administration—the keepers of all government records—manages millions of digital records. But users have trouble finding the records they’re looking for, and the current manual metadata tagging processes aren’t sufficient.
The agency recently held a virtual informational day outlining its goals for integrating artificial intelligence and machine learning into two ongoing projects: personalizing the catalog search function and automating metadata tagging.
The archive’s catalog currently holds more than 120 million digital records, as well as “archival metadata and other types of records, including electronic databases.” However, the system has “an unsophisticated search” function, according to a request for information.
While NARA employees add metadata tags to digital records, “There is a delta between what NARA has been able to describe and the specific information that users want from our records,” the RFI states, asking, “Can AI fill the gap?”
During an informational day held in early April, NARA executives outlined some of the challenge, including a single search returning a flood of results from the same source—making it difficult to sift through to find multiple sources—and difficulty distinguishing between records with similar names, such as a search for “Truman” the president versus “Truman” the aircraft carrier.
The current search function also is not able to return accurate results if the search term input is not exactly the same as it exists in the metadata.
The RFI is seeking feedback on automated solutions that can analyze how users search the digital archives and associate those search terms with the appropriate record.
This effort is also looking at ways to customize the search experience for returning users.
“Can we customize the experience so that the user gets to what they want more quickly? What tools can we use to improve the search experience for the user without requiring additional manual work from our staff?” the RFI asks.
In a similar but separate line of effort, NARA officials are also looking at ways to automate the metadata tagging process to move away from relying on employees to manually tag records.
“To make digitized holdings accessible to users in the catalog, archival descriptions—metadata—must be manually entered by NARA employees prior to being uploaded,” the RFI states. “AI/ML technologies that could automate the creation of these required fields—and possibly more fields than we currently have—could greatly increase the accessibility of digitized holdings.”
The ideal solution would identify useful metadata at the point of ingest—when agencies transmit data to NARA for archiving—and automatically apply those tags as the records are archived. At that point, incoming records would be “self-describing,” rather than relying on manual descriptions.
Both of these efforts focus on answering three key questions:
- How can NARA make records easier to find?
- How can NARA make records available more efficiently and quickly?
- How can NARA ensure its data’s integrity?
The RFI seeks to answer those questions while investigating the best technical solution and acquisition strategy, including:
- Identifying and addressing data quality issues such as bias.
- Anonymization versus personalization of user-friendly search.
- Algorithms, frameworks and tools for creating AI solutions for the use cases, indicating outcome, addressing the strengths and limitations.
- Comparison of capabilities provided by different cloud providers such as AWS, Azure, IBM, Google, etc.
- Commercial off-the-shelf or cloud-based versus non-cloud based tools or frameworks.
- Related licensing costs, operating capabilities and required support.
- Storage and indexing capabilities agnostic to data format and type.
- Design of pipeline for development and delivery of AI and ML solution to production.
- Post-production activity needed such as infrastructure monitoring, debugging, job orchestration, etc.
- Areas for cost considerations.
White papers addressing the use cases are due by noon on May 10. After reviewing those submissions, NARA officials might opt for virtual follow-up meetings, which would be scheduled after June 9.