3 Keys to Full Spectrum Data Management
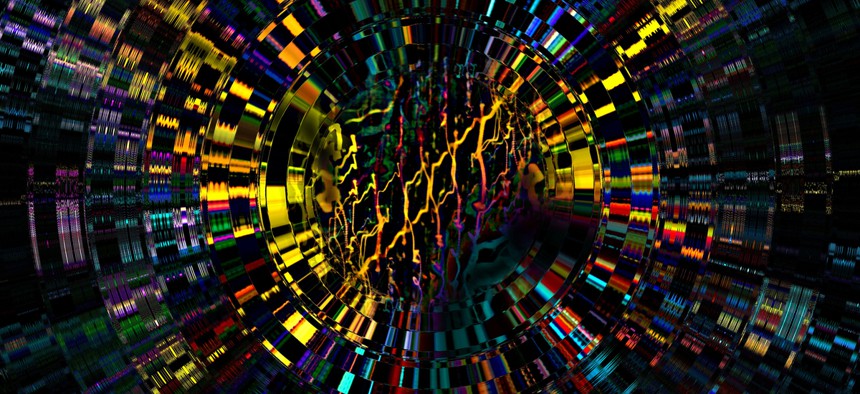
marymyyr/Shutterstock.com
Full-spectrum data management means having a grasp on data across complex systems composed of private and public clouds, edge devices, and legacy applications and infrastructure.
The internet of things has, to put it bluntly, blown the walls off the data center. A tremendous volume of data is being generated by connected devices, and it’s widely dispersed. It’s also critical to stakeholders. In 2019, public sector chief information officers and chief technology officers leaned into a multi-hybrid cloud strategy. This year, they’re trying to manage the data diaspora and volume that comes with it.
Full-spectrum data management means having a grasp on data across complex systems composed of private and public clouds, edge devices, and legacy applications and infrastructure, while also ensuring its secure and data management process are compliant.
Often, conversations center on whether to move everything from the edge to the data center or, conversely, to push analytics and inferencing to the edge. In reality, full-spectrum data management relies on both, including the ability to automatically decide which approach is appropriate. Let’s look at three key components of full-spectrum data management.
Managing Metadata
Overall, a full spectrum data management strategy is about standardizing and simplifying as much as possible—and the first tool to that end is a federated metadata manager. This allows metadata to be stored and accessed from myriad locations on demand via a common management layer and stands in contrast to a traditional centralized data management structure, which cannot scale or be readily available.
One benefit of a federated metadata manager is that it prevents unnecessary copying and transformation, which can help keep data volumes down. Far too many organizations are terrified to throw data away, so they store multiple copies in different formats. But exabytes of data are being generated at the tactical edge. Multiply that by three or four because of copying, and data volumes become untenable. With metadata management, you know how to access raw data from anywhere and only transform it when it’s absolutely required.
Data Blueprints
Of course, it’s impractical for every application to deal with raw data completely from scratch. A data blueprint is essentially a template that can be applied to any different data stream coming in. It’s a playbook that can be used repeatedly with only minor modifications. Every application likely wants to use raw data in a different way, so the blueprint should populate the metadata management layer with appropriate tags and ways to access the data.
Consider, for example, the automotive industry. Canned data from the car’s sensors include everything from temperature to tire gauge pressure—but it’s slightly different for each car. A data blueprint would know how to process the incoming data in a general way. For each specific car, the template would simply have to be tweaked. When the data is brought into the data management layer, the blueprints then become discoverable via the application at hand. The application can reach down into the metadata management layer and bring back only the data it needs—temperature data, perhaps, to make a weather prediction.
Data Orchestration
Finally, full-spectrum data management means that service, infrastructure and data orchestrators work together to decide when data should be moved—and to do so automatically when that’s the case. Let’s say, for instance, that a terabyte of data has been marked as classified. Policies in the data orchestrator should prevent movement, telling the service orchestrator to bring the container to the data instead. Basic data orchestration rules may limit movement based on size—particularly useful so no one accidentally burns through their public cloud budget due to poor decision-making—but more sophisticated considerations included classification, compliance, regulation, and health.
Usually, when someone develops an application, they must determine upfront whether it will run on the edge or in the data center—and must essentially write a mini orchestrator themselves to that end. The goal of full-spectrum data management is to that responsibility out of the hands of the application developer so a middle layer can instead make the call. This enables developers to write applications that can run anywhere and that can automatically coordinate between the edge, data center or cloud.
The Bottom Line
Especially in the public sector, solutions are deployed in an extremely dynamic environment. What can be done on the edge today may not be plausible tomorrow. Thus, you need an underlying data management plan—one that knows data locality, lineage, classification, and governance—in order to allow for sufficient flexibility, such as moving a container to the data or allowing an application to rapidly access metadata tags.
For public sector executives, achieving the next level of data management simply must be top priority. Full-spectrum data management means effectively handling data that’s spread all over the place—something that’s particularly crucial as the internet of things multiples the volume of data organizations rely on.
Darren Pulsipher is Intel's Chief Solutions Architect for Public Sector.