The Promise and Limitations of AI in Cybersecurity
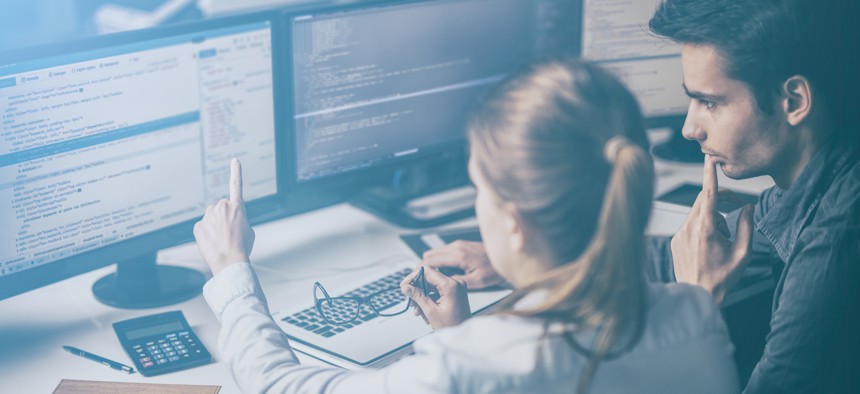
REDPIXEL.PL/Shutterstock.com
In the cybersecurity arena, hype runs deep, and AI is no exception.
Artificial intelligence, once seen as a dead end in computer science research, has surged to the fore in recent years. New technologies built on neural networks, machine learning, and deep learning, combined with virtually unlimited MIPs and storage in the cloud, have started to show promise for solving certain kinds of problems. In many cases, the promise is justified. But marketing hype makes it difficult to distinguish the real applications of AI from the bogus ones.
In the cybersecurity arena, hype runs deep, and AI is no exception. Most chief information security officers of larger entities are intrigued by the promise of AI but skeptical when they see vendors touting AIs that can detect and neutralize threats without high false positives. They know that AI-based cybersecurity solutions require a close partnership between humans and machines.
The Paradoxical People Problem
Instead of eliminating the need for security staff, AI solutions actually require dedicated staff to manage them. You need people to train the AI and tune its output in order to ensure that its recommendations are as useful as possible. You also need people to monitor AI-generated alerts to determine which ones are real threats and which are false positives.
A recent Gartner survey found that AI was the most-often-mentioned cybersecurity technology that CISOs are considering. But, if you look closer at this study, it becomes clear that CISOs are experimenting with AI, not installing it in mission-critical applications. In fact, the analyst who conducted the study warned CISOs to be prepared for disillusionment.
For that reason, smart CISOs view AI as a component of a layered security infrastructure, also known as a defense-in-depth strategy.
The Uses and Limitations of AI in Cybersecurity
If you have a large set of data with divergent characteristics, a well-trained AI system can throw it into relevant buckets. For instance, AI is good at identifying spam in email messages, because spam looks nothing like legitimate content.
AI also works well at anomaly detection, provided that you can easily validate the intent behind a potential anomaly. For instance, credit card processors use AI to flag potentially fraudulent transactions. It’s not that the technology is particularly accurate at identifying fraud—there are a lot of false positives, as anyone whose card has been blocked because they shopped at a new store knows. The reason this approach works is because it’s relatively easy for the credit card issuer to ask if you really meant to buy those two plane tickets to Aruba or not.
Where AI runs into trouble is when the threats are virtually indistinguishable from the legitimate activities (like a BEC attack, which looks almost exactly like a real message from your boss), when false positives are difficult or expensive to investigate and verify, or when you don’t have a clearly definable baseline of what “normal” data should look like.
Of course, AI also works best with huge volumes of data. In an era when people and governments are increasingly concerned about the leakage of personally identifiable information, that may limit the application of some types of AI cybersecurity.
Combining AI With Other Defensive Technologies
These limitations are why AI needs to be combined with other technologies in a layered defense. To be effective, these other layers should not rely on the same kind of techniques as the AI layer.
For instance, while your AI is focused on content analysis and network activity monitoring, other layers can address identity, authentication and access control. If you can verify that the people and applications accessing your network really are who they say they are, then AI can focus on identifying the obvious break-ins or cases where someone’s password has been compromised.
Or, if you validate that every domain and service sending email into your inbox is trustworthy then AI can focus on flagging the comparatively rare cases when a legitimate email sender has been taken over by a hacker delivering malware. The combination of these techniques is vastly superior to any single approach.
In short, AI has potential. But it only addresses specific attack vectors and depends heavily on the people who have to train and refine it. The best way to deploy AI is to consider it as one of several defensive layers and balance it with a variety of different approaches.
Alexander García-Tobar is chief executive officer and co-founder of Valimail.
NEXT STORY: It’s Time To Make Data Strategic For Our Navy