Nothing Pixelated Will Stay Safe on the Internet
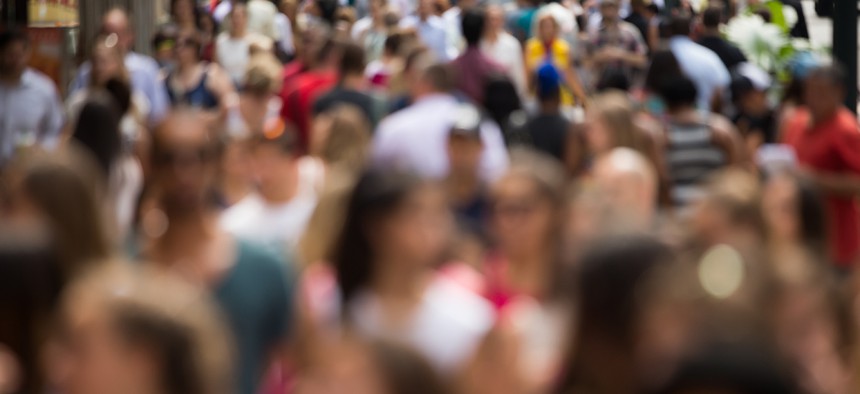
blvdone/Shutterstock.com
Sensitive information such as obscured license plate numbers or censored faces may soon become easier to reveal.
It’s becoming much easier to crack internet privacy measures, especially blurred or pixelated images. Those methods make it tough for people to see sensitive information such as obscured license plate numbers or censored faces, but researchers from University of Texas at Austin and Cornell University say the practice is wildly insecure in the age of machine learning.
Using simple deep learning tools, the three-person team was able identify obfuscated faces and numbers with alarming accuracy. On an industry standard data set where humans had 0.19 percent chance of identifying a face, the algorithm had 71 percent accuracy (or 83 percent if allowed to guess five times).
The algorithm doesn’t produce a deblurred image—it simply identifies what it sees in the obscured photo, based on information it already knows. The approach works with blurred and pixelated images, as well as P3, a type of JPEG encryption pitched as a secure way to hide information.
Specialized tools for seeing through blur and pixelation have been popping up throughout this year, like the Max Planck Institute’s work on identifying people in blurred Facebook photos. What distinguishes the UT and Cornell research is its simplicity. The attack uses Torch (an open-source deep learning library), Torch templates for neural networks, and standard open-source data.
“We’re using this off-the-shelf, poor man’s approach,” says Vitaly Shmatikov, co-author of the paper and professor at Cornell. “Just take a bunch of training data, throw some neural networks on it, throw standard image recognition algorithms on it, and even with this approach … we can obtain pretty good results.”
Shmatikov acknowledges the Max Planck Institute’s work is more nuanced, taking into account contextual clues about identity. But he says his simpler approach shows how weak these privacy methods really are. (He doesn’t mention his method also is 18 percent more accurate in a comparable test.)
To build the attacks that identified faces in YouTube videos, researchers took publicly-available pictures and blurred the faces with YouTube’s video tool. They then fed the algorithm both sets of images, so it could learn how to correlate blur patterns to the unobscured faces. When given different images of the same people, the algorithm could determine their identity with 57 percent accuracy, or 85 percent percent when given five chances.
“It’s pretty simple stuff,” says Richard McPherson, co-author and a visiting student at Cornell Tech. “The only real restriction is having a data set you could train these machine learning techniques on. But that’s available.”
Training data could be as simple as images on Facebook or a staff directory on a website. For numbers and letters (even handwritten), the training data is publicly available online.
Companies like YouTube that recommend blurring should make it clear their privacy measures only protect information from humans, not machines or determined adversaries, say McPherson and Shmatikov.
“In security and privacy, people do not fully appreciate the power of machine learning,” Shmatikov says. “Until somebody shows how even off-the-shelf technology can be used for privacy breaches, people in security and privacy aren’t going to realize it.”
NEXT STORY: New policy on encryption appears unlikely