IARPA grapples with threats posed by generative AI
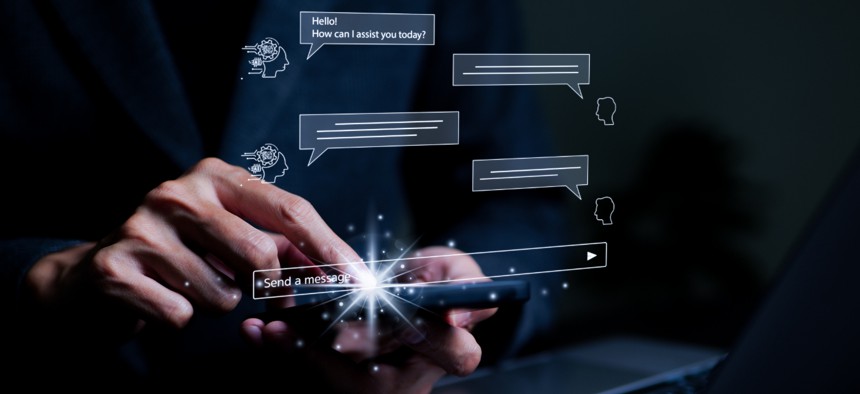
Supatman/Getty Images
The intelligence agency is looking for insights about the vulnerabilities within large language models and how it can prevent false information from harming the analysts using them.
The Intelligence Advanced Research Projects Agency wants to understand how the large language models that help fuel artificial intelligence and machine learning chatbots are vulnerable to threats and biases.
IARPA officials issued a sources sought notice Monday to gain more insight into what threats and vulnerabilities could be inherent in LLMs, how they are characterized and what strategies could be used to mitigate them.
LLMs are machine learning models that compile data on human languages and can mimic a neural network to help generate text-based responses, such as OpenAI’s ChatGPT. LLMs can receive inquiries from a user and pull data to help generate responses that seem like they were delivered by a human.
But the challenge with LLMs is that, while their responses may appear authoritative and even conversational, their actual knowledge is limited to the amount of language text consumed in their training datasets, possibly generating misleading or inaccurate responses.
According to the RFI, IARPA is examining the potential use of LLMs in working with intelligence analysts, but the agency is also exploring what frameworks could be established to offset the potential errors and vulnerabilities of the technology.
“LLMs have received much public attention recently due, among other things, to their human-like interaction with users,” the notice said. “These capabilities promise to substantially transform and enhance work across sectors in the coming years. However, LLMs have been shown to exhibit erroneous and potentially harmful behavior, posing threats to the end-users.”
Specifically, IARPA officials want to know what frameworks exist to classify and understand the range of LLM threats and vulnerabilities, how those threats are identified, what methods may be in place to mitigate those threats and how confidence in LLM responses might be quantified.
The RFI calls for how those frameworks might apply to both white box LLMs that have privileged access to their code and black box models that do not.
Interested stakeholders have until 5 p.m. EST on Aug. 31 to respond.