3 guardrails for sustainable AI implementation in the public sector
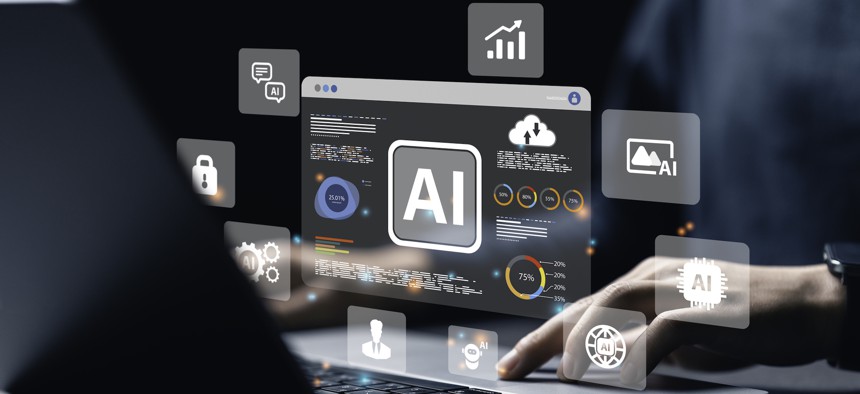
Khanchit Khirisutchalual/Getty Images
COMMENTARY | Starting with low-risk use cases can help build best practices while avoiding more significant pitfalls such as data leakages and security vulnerabilities.
AI is poised to transform how public sector agencies approach mission-critical operations and solve the government’s greatest challenges. It can identify waste and fraud in government, strengthen cybersecurity efforts, and power chatbots and other tools to better serve constituents. However, because AI is an emerging technology, the government needs to understand the associated benefits and risks to maximize its opportunity.
The government has taken action to harness and support AI innovation through resources like the National Artificial Intelligence Research Resource Task Force’s AI implementation plan, the NIST AI Risk Management Framework, and the White House's AI Executive Order. Many of these resources are focused on providing agencies with a framework to advance responsible AI, an ideal that’s only possible if agencies understand what’s in the systems, software, and datasets they’re utilizing.
The public sector can leverage the various capabilities of AI but has the added pressure of maintaining constituent trust with every step. Agencies must develop implementation strategies that proactively address constituent concerns around AI’s accuracy, reliability, and security. Let’s walk through three critical steps for the sustainable implementation of AI in the public sector.
Identify low-risk AI implementation areas
Rather than starting with an overhaul of an agency’s entire technological infrastructure, organizations should begin by applying AI to a low-risk use case, and build from there. For example, agencies can start using AI to analyze legacy applications to provide developers with an automated explanation of the code and how to modernize it. This can help build best practices that can be applied across the organization as the rollout progresses while avoiding more significant pitfalls such as data leakages and security vulnerabilities.
To begin the process, IT leaders should facilitate conversations between their technical, security, and legal teams, as well as their AI service providers to set a baseline of agreed-upon goals, determine key focus areas, and identify any potential risks. Then, agencies can begin implementing guardrails for AI implementation such as employee-use data restrictions, in-product disclosures, and moderation capabilities. Agencies should also develop policies that ensure their data is ethically sourced and free of any biases that could infiltrate their AI models.
Identifying a low-risk AI implementation area to start, followed by building best practices and policies for its use, allows organizations to safely and strategically benefit from the efficiencies of AI, while also ensuring it can safely scale.
Partner with transparent vendors
When selecting AI vendors, organizations should ask three primary questions: First, what data are the AI models being trained on, and how was it collected? Second, will the organizations’ data be used within these models? And finally, will their data be retained by the vendor? These questions provide a baseline for transparency when choosing an AI vendor.
Agencies can more confidently implement generative AI and other AI functions if they know that their intellectual property, like source code, or other data such as personally identifiable information, will remain within their control, and will not be used to train other AI models. The integrity of an agency’s systems and underlying data must adhere to a chain of custody to be trusted, making it nearly impossible to implement AI safely and responsibly without a similar chain of custody applied to the AI models and training data being used.
The more transparent a vendor is, the more informed an organization can be when assessing the partnership. Agencies have a responsibility toward their employees and constituents to ensure that they are implementing AI responsibly. Through transparency, vendors have an opportunity to help agencies securely adopt AI and implement security and privacy best practices without sacrificing adherence to compliance standards–and ultimately, risking constituent trust.
Protect constituent data with contingency plans
Finally, agencies must implement security policies surrounding the use of AI. Without guardrails, organizations risk compromising sensitive data and threats to national security. Reviewing how AI services handle proprietary and customer data, including the storage of data shared with and received from AI models, lays the groundwork for scalable, sustainable AI adoption.
Accountability is critical to maintaining trust with constituents. Government agencies are held to security and compliance standards that reinforce trust. By understanding how data is collected, transacted, and stored, agencies can proactively protect their infrastructure against threats to data privacy. Agencies and their partners must take a privacy-first approach to protecting user information and intellectual property by containing it, and its source code, within internal IT environments.
Emerging technologies like AI present new challenges, and their evolution can be hard to predict as agencies are at the earliest stages of utilizing these new capabilities. By following a framework that includes guardrails around AI adoption and use, agencies will be proactively positioned to understand its challenges and vulnerabilities as they emerge, and safely leverage AI’s many possibilities.