NIST promotes testbed to address threats targeting ML systems
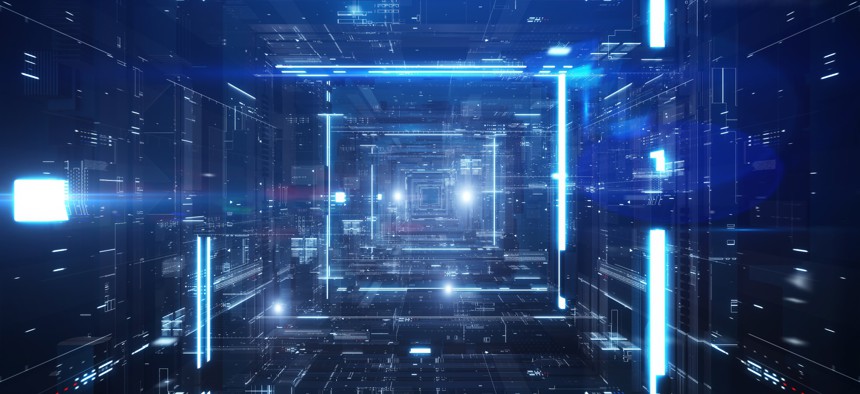
Getty Images
Dioptra, an experimentation testbed fielded by the National Institute of Standards and Technology, allows researchers to better understand the techniques that can help protect their products and systems against an increasing number of attacks targeting machine learning algorithms.
The National Institute of Standards and Technology is promoting an experimentation testbed to address the changing cybersecurity landscape and increasing threats targeting machine learning algorithms, offering researchers a new way to test products against a range of attacks.
The National Cybersecurity Center of Excellence, a public-private sector collaborative hub within NIST's Information Technology lab, showcased the first iteration of the testbed, called Dioptra, at the Information Security and Privacy Advisory Board (ISPAB) meeting on Wednesday.
Harold Booth, a NIST computer scientist and project lead for the National Vulnerability Database, described Dioptra as a method of evaluating which security techniques and solutions can best protect ML-enabled systems, allowing researchers to compare methods against a diverse set of attacks, datasets and other various conditions.
"The basic take-home message is that there are a lot of metrics and measurements out there, but you really want to give some thought to what [solutions] you're using and make sure you understand how those play with your deployments," he said.
The testbed follows NIST Internal Report 8269, a taxonomy of adversarial machine learning that the agency published in 2019 which identifies three major categories of attacks against ML algorithms: Evasion, poisoning and oracle. Such attacks manipulate or alter test data to cause ML models to behave inaccurately, or reverse engineer models to benefit the adversary.
Researchers can use Dioptra to test multiple combinations of attacks, defenses and model architectures within their systems to better understand which attacks may pose the greatest threats, and what solutions may keep their ML algorithms the safest compared to numerous techniques.
As part of the Dioptra risk assessment process, NIST recommends researchers identify threat and deployments assumptions, determine which attacks are relevant to their products, build experiments and synthesize results, and identify metrics applicable to the highest priority risks. The agency also poses the question of whether deploying AI and ML is necessary within the specific system or product they are developing.
As NIST continues encouraging federal agencies to use AI and ML within their systems, the agency envisions the machine learning security testbed to serve an expansive audience of researchers, analysts and first-party developers in order to build cybersecurity testing into each phase of product development. Eventually, NIST says the testbed may even serve consumers who wish to verify the assessment process for various ML products.