How to prompt better responses from private, federal ChatGPTs
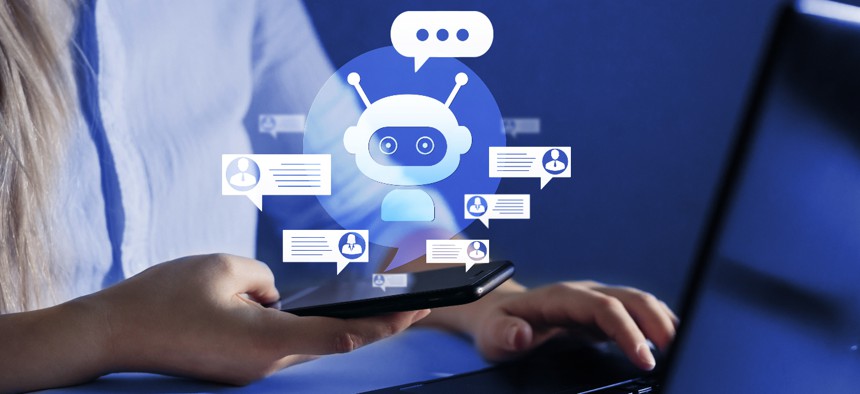
Galeanu Mihai/Getty Images
Problems with mini-GPTs often come down to poor prompt engineering when they were originally created.
Back in December, I experimented with the newly-enabled ability to customize mini-GPTs for use with specific tasks, a feature that was added to the main ChatGPT site, which is the home of one the most advanced AIs available. After spending quite a few weeks learning how to build smart, responsible and effective mini-GPTs that conformed to all federal guidelines, including the Executive Order on responsible AI use, Nextgov/FCW published my guide to help federal agencies build their own mini-GPTs. The idea was to enable feds to unlock the power of generative AI without bringing along many of the most common drawbacks, like biased results, incorrect or nonsensical answers, or outright AI hallucinations.
And from the volume of email and direct messages on the X social media platform that I received, it seems like a lot of people gave mini-GPT creation a try. And while some people achieved good results, including creating AIs that only used federal data, many also expressed disappointment at the performance of their creations. Some even said that their custom AIs were not very smart at all.
I contacted quite a few of the people who wrote me and talked with them about where their federally-focused AIs were going wrong. Sure enough, some of the results they shared with me were pretty off-the-rails. A few were almost comically bad. And many of those mini-GPTs were even trained exclusively on federal data sets, so, at first glance, it would seem like they should have worked well. But the problem with most of the ones I examined came down to poor prompt engineering when they were originally created.
Prompt engineering is truly an emerging science. It involves writing very specific queries and training an AI to perform exactly as expected. It basically boils down to knowing how to “talk” to an AI in a way that the artificial intelligence understands, so it can provide the answers you want, and does not get confused when executing commands. Top prompt engineers can earn as much as $300,000 or more per year right now, but one day they may not be needed at all, as the interfaces with AIs also slowly improve and become more human-like. But for now, good prompt engineering is a key to success, and also what seems to be the main reason why so many of those customized federal Chat-GPTs that people wrote to me about are failing.
And while prompt engineering is often thought of as the questions that users ask an AI, in this case good prompt engineering is needed in order to initially create a mini-GPT so that it acts correctly. Instead of relying on users to write good prompts, you can set exactly how a customized GPT will act, and how it will respond to users. If you do that part really well, there is less chance that sloppy or vague user queries will return odd results.
How to write good AI prompts
If you refer back to my original guide, writing good prompts was the second step when creating a mini-GPT. Remember that generative AIs will naturally try and guess what a user wants to hear next, which historically was their original function. So, if you are not very specific about how you want the mini-GPT to act, it will likely make something up in terms of its behavior, and that will often be wrong.
When setting the terms of how your mini-GPT should perform, it’s important to be very specific about what you want it to do. For example, for a GPT focused on streamlining procurement, don’t tell it to simply analyze proposals given to it for government suitability. That’s too vague, and gives the AI too much room to add in irrelevant information in its responses. Instead, tell it to analyze individual proposals and evaluate them against specific federal guidelines and regulations, and then provide any instances where proposals are not in compliance.
Another good prompt to use is to require the AI to cite its sources for every response. This can be especially helpful if you have fed it only federal documents and information, because it can easily show which ones that it’s basing responses on, something that the main ChatGPT almost never does natively.
You might also consider defining the format that your mini-GPT will use in its responses to help limit errors even further. This can be as general as telling it to limit replies to no more than 100 words or a couple of sentences, or as specific as asking it to tailor all responses into specific, government forms.
This type of response-defining prompt can also be used to limit the biased responses that some users were experiencing with their customized GPTs. For example, your prompt can tell the mini-GPT that instead of providing users with a judgment call about which proposal or document is better, that it should instead generate a list of pros and cons — and cite its sources for each of those decisions. This backend prompting can override poor user prompts too, helping to make the mini-GPT much more specialized, although some user training is always helpful.
Finally, while this is not prompt-specific, one of the other problems that federal users were having was their mini-GPTs not realizing the context or the importance of the documents it was fed to train on. Again, referring to our original guide, uploading approved government documents for the GPT to learn is done during step two, specifically by clicking on the Knowledge tab. It took me a while to figure this out, but most users who were having a lot of trouble had shared relevant documents with their mini-GPT without providing any context, or at least not enough context.
Whenever you upload a document that will act as the baseline for a mini-GPT’s decisions, the interface prompts you to explain what it is. This is not an inconsequential step and goes far beyond just labeling the content. You really need to define what each document means, and how important or weighty the information contained within it is when applied to the GPTs core function. For example, in the case of Section 508 regulations, instead of just telling the GPT what section 508 is when feeding it information, it’s important to explain how meeting those requirements is critical for all relevant procurements. Tying that into the previous prompt requiring a mini-GPT to generate pros and cons for each response, you could tell the AI that when it finds any proposal that does not meet the standards of the 508 regulations, it should immediately generate a “con” at the top of that side of the list, including citing the specific standard that is out of compliance.
Better AI through prompting
I am happy to report that most of the people I worked with over the past few months that were experiencing uneven results with their mini-GPTs were able to see a lot of improvement by tightening up their prompts and better defining the goals of their federalized AIs. As advanced as generative AIs are, using them is still very much a learning process in a lot of ways, and the key may be trial and error coupled with a good deal of patience. But in general, using very specific prompts and tightly defining what an AI should do and how it responds are the best ways to ensure a high level of accuracy.
And if you don’t feel like diving into all of that, there is now a growing marketplace of already-programmed mini-GPTs to choose from. OpenAI features top-performing mini-GPTs each week in a variety of categories from a rapidly expanding pool. Most are not going to be very helpful for the federal government, but a few might be worth checking out if there is a very specific need that could use a little bit of assistance from a well-programmed and prompted AI companion.
John Breeden II is an award-winning journalist and reviewer with over 20 years of experience covering technology. He is the CEO of the Tech Writers Bureau, a group that creates technological thought leadership content for organizations of all sizes. Twitter: @LabGuys