Why AI localization makes sense
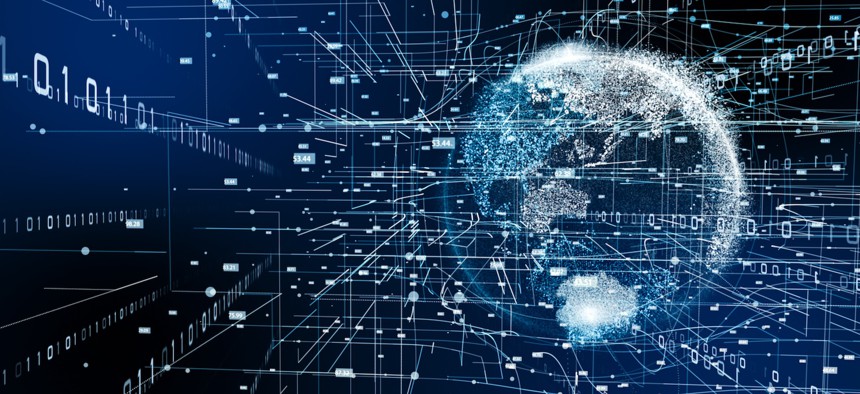
Tony Studio/Getty Images
COMMENTARY | Localization supports faster response times and more intelligent interactions between users and systems.
Artificial intelligence is expected to dominate government technology in 2024, especially on the heels of the Biden administration's publication of the Executive Order on Safe, Secure, and Trustworthy AI. Another topic that will garner attention and become a recurring concept is the process of training AI with localized data, also known as AI localization.
As of December 2023, there were 1,200 reported current and planned AI use cases across federal agencies. That number will likely increase this year as federal departments, such as the Department of Justice, hire chief AI officers to lead and carry out AI-focused objectives.
Federal agencies continue to explore the benefits of AI technology across the government, and as adversarial threats emerge, it’s more important than ever for agencies to adopt intelligent data collection practices that align with privacy guidelines and industry regulations. After all, AI is only as accurate as the data provided and the data it is trained on, and not all data is universally accurate.
By training AI on localized data, government leaders can tailor systems and accommodate diverse cultures in different markets, combining natural language processing and machine learning. By utilizing this method of AI training, federal agencies can significantly improve citizen services and experiences with faster response times, higher resolution interactions and more accurate communications. In turn, government can bolster its trust with citizens and enable ethical, timely outcomes through the proper use of AI technology.
Understanding localized data
Localized data is a form of data that incorporates various linguistic, cultural and demographic sources. It creates an environment where privacy is paramount, keeping data in one central area that can be used for AI or ML processes.
AI localization is not to be confused with data localization -- a data storage strategy designed to support compliance with national data privacy laws. AI localization is designed to generate AI outputs that are more useful and understandable to specific populations.
When localized data is used to train AI models, the output produces more accurate, ethical and fair results, enhancing the solution or service the data is being used for. For example, if an agency providing healthcare to citizens trained its AI model on localized data to predict health outcomes for citizens in the Midwest, they would likely get very different outcomes if they trained the same system with health data from those on the West Coast due to stark diet and lifestyle differences.
By adopting the practice of training AI models with localized data, CAIOs and other government AI leaders are displaying their commitment to ethical systems and fostering a culture of inclusivity across their respective agencies. At the same time, they highlight the importance they’re placing on security by using data that can be controlled and collected in a single source, ensuring that all data used is from a single location.
As a result, agency employees and officials can gather a more holistic and enhanced understanding of the citizens they serve based on the data created from the localized AI outputs.
Impact on federal operations and CX
Training AI with localized data benefits agency operations in several ways, including enabling privacy compliance, improved response times, clearer communications and more.
Since AI success is based on ethical data collection, agencies implementing localized AI models will be assured their models comply with outlined privacy requirements – 75% of all countries have implemented some level of data localization rules, with one of the most significant reasons being to address rising concerns about privacy. Since this type of data collection combines real-time and model data, the outcomes are aggregated and adhere to a high level of confidentiality, ensuring citizen identities are not at risk of being identified.
In addition to privacy compliance, localized AI systems enable localized solutions to serve citizens with unique needs. Tailoring ML and NLP models is not only a technological advancement but also ethically imperative.
One such example would include training AI chatbots using different languages to ensure the citizen’s concern or inquiry is understood and addressed correctly. If such tools are only trained in the English language, a significant number of the nation’s citizens risk being misunderstood and unsatisfied with the services provided by the government.
As AI continues to evolve, there is also room for improvement with localized data beyond the above. Furthermore, federal leaders can refine the data to include regional offices and constituents. In this case, courts with different regional/circuit court jurisdictions will be provided with more tailored outcomes as their interpretations and regulations differ.
Improving how AI models are trained is undoubtedly a technological advancement. Still, even more, it is seen as significant ethical progress, which is required to best serve all citizens with the resources and services they rely on daily.
NEXT STORY: Checking in on digital government in China