What the Government Can Learn from Netflix
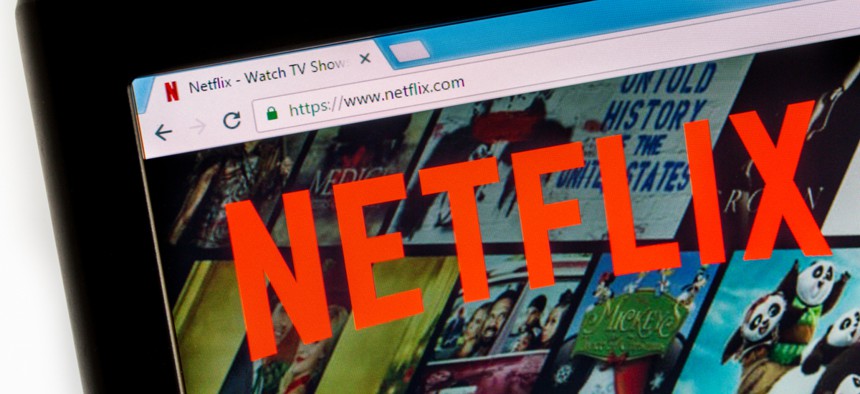
pixinoo/Shutterstock.com
Customers have moved past the search engine.
At the turn of the 21st century, a search engine was the most valuable tool for finding what you needed on the internet.
Websites such as Netflix, eBay and Amazon grew due to their powerful and persuasive search engines that enabled users to freely mine site content and discover solutions to their wants and needs. However, this simple “find what I’m looking for” capability that was considered so valuable now seems almost antiquated.
A search engine works reactively, only producing results when the user requests something. In contrast, a recommendation engine predicts what user might want and preemptively provides results that aid in a discovery process. A recommendation engine presents relevant content that users did not necessarily search for or of which they might not already be aware.
Recommendation engines are a branch of information retrieval that uses artificial intelligence. These engines provide powerful tools and techniques to analyze volumes of data—especially product information and user information—and are designed to correlate with user profile themes and characteristics and then provide relevant suggestions. In technical terms, a recommendation engine is a mathematical model that can predict how much a user will prefer an item. A key difference from the search engine is that the underlying goal is not to sell more but to learn more about prospects and then offer a great recommendation that becomes a catalytic precursor to insight. In short, recommendation engines are automated "hypothesis recommenders" that identify correlations which might merit real-world exploration. And they can be used in many sorts of virtual interactions beyond a retail sale.
We think it is time for government agencies to embrace the potential of recommendation engines. As an example, consider a competitive grant maker such as the National Science Foundation. NSF conducted more than 240,000 reviews in 2017 of about 50,000 grant application proposals. Every proposal received includes a written abstract. NSF staff managing the proposals often spend considerable time finding suitable reviewers for the application by looking through CV databases, academic credentials, availability and history. But what if NSF’s Grants Management System could automatically "recommend" reviewers for grant applications by understanding proposal abstracts and correlating the abstracts to reviewers’ background and work history? Such a capability could considerably decrease the time to find, assign and complete the peer review process.
Similarly, consider the Food and Drug Administration’s imports processing system. FDA electronically screens foreign-made food, drugs and medical devices before they enter the U.S. There were about 40,000,000 product lines imported into the U.S in 2017. FDA uses PREDICT, a rules engine that forecasts issues in import lines using data from several sources, such as U.S. Customs and Border Protection’s IT systems, FDA’s own inspection results, and inherent product risks (i.e., spoilage of perishable food or drugs). The rules-based system employs proprietary coding developed by a contractor that supports PREDICT. Changes in that programming to accommodate revisions to regulations can be costly. Instead, an AI-based recommendation engine could predict issues in both new and existing import lines without needing explicit programming or rules definition by reviewing imports and inspection data, historical data for similar products, and compliance data, then recommending import lines most likely to have issues and require inspections.
Another federal activity that could benefit from recommendation engines is the procurement and grant-making function. Government agencies collect and report data on federal procurements and grants through the Federal Procurement Data System and the System for Awards Management. Using past performance and capability statements, plus bids, proposals and grant applications received, a recommendation engine could identify suitable vendors that the agency should invite to compete for a contract or other award. This might dramatically reduce the market research effort needed by acquisition staff.
While there are many benefits to recommendation engines, building a good one poses challenges to all the actors of the system. For example, deep learning algorithms cannot provide a rationale for any particular recommendation. Since government agencies favor transparency, we suggest supplementing deep learning with machine learning techniques that can offer a better rationale for the recommendations. From a government perspective, transparency is essential to seek relevant suggestions from a trusted source for decision making. So, the recommendation engine needs to be built in such a way that it ensures the confidence of its users. From a data perspective, organizations should evaluate their data strategy to ensure that they can systematically organize and access vast amounts of structured and unstructured input and output data.
To conclude, government organizations should explore AI-based recommendation engines by understanding and investing in AI technologies and techniques, and considering their implications, challenges, and constraints. AI capabilities, uses and experience are rapidly evolving, thus agencies may want to identify a trusted partner to help develop the infrastructure and processes needed to explore the potential of AI.
Sanjeev Pulapaka is a solution architect and Srikanth Devarajan is an enterprise architect at REI Systems.