6 ways for government CDOs to succeed
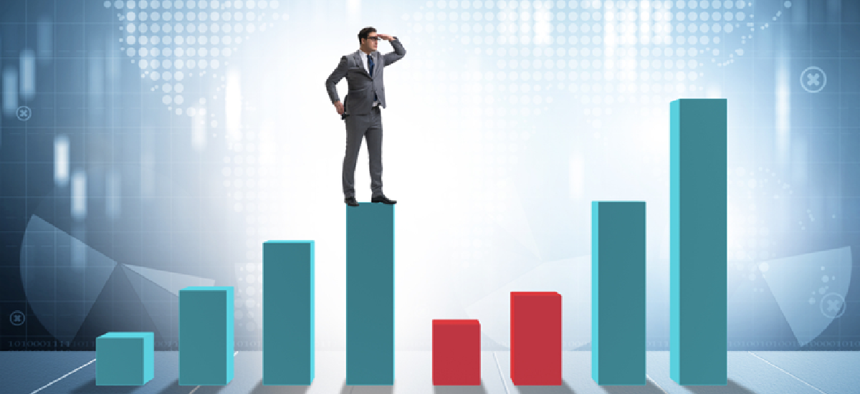
The government agency chief data officer role comes with nuances, and to ensure success, officials need to be mindful of far more than simply structuring datasets.
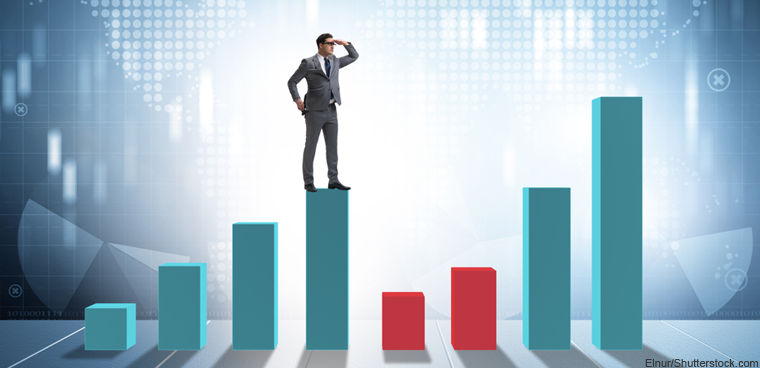
From as early as 1790 when the first U.S. census occurred, the United States government has pioneered the collection and distribution of data. This longitudinal dataset that spans from 1790 through 2010 is one of the longest standing datasets and is frequently used by both government and businesses to perform an incredibly wide array of analyses.
In the 21st century, government data plays a major role in everything from forecasting presidential elections to scheduling road maintenance to fighting poverty. Watching as data flows in precinct-by-precinct during an election, for example, allows higher transparency and engagement by the electorate and enables data scientists to analyze patterns and trends for future marketing or detect anomalies to prevent fraud.
While these examples show how data science can make a powerful impact on critical decisions in the public sector, it is becoming increasingly intricate and challenging. The majority of government data is difficult to find, get clearance to use and wrestle into a form that is useful. To help ease the complexity of this issue, the coming Federal Data Strategy will guide agencies to meet a new mandate to bring on a chief data officer (CDO) whose role will be to help prioritize the dissemination of data to improve internal government use across agencies, as well as use of government data by the public.
The Federal Data Strategy is an exciting prospect for CDOs and data scientists, but to ensure they are delivering the most value possible, there are several things these data strategists need to consider.
1. Leverage best-in-class models for sharing
While many public and private organizations have set up data repositories for sharing useful datasets across diverse user groups, many repositories are difficult to use, hindering data sharing and collaboration. The power in data science is multiplied when others can contribute. It is crucial for CDOs to build repositories with models that allow other parties, especially local and state agencies, to contribute their own datasets to federal datasets. With access to this collaborative repository, data scientists can analyze wider, more holistic trends and glean even more valuable insights to inform decision-making.
It is also important that CDOs enable data scientists to conduct analysis of large collections of data quickly and accurately. To do that, the CDO will need to build models that see data profiles instantly and format universally. For example, identifying the number of data fields, the value distributions or the number of nulls is significant information when analyzing data, especially when the analysis needs to be done collaboratively. It will be the responsibility of the CDO to ensure repositories are set up for successful analysis.
The diverse data that exists in the public sector is unique and requires CDOs and data professionals to leverage best-in-class models that allow for collaboration. These datasets in particular have the potential to drive decisions that impact some of the nation's biggest challenges.
2. Diversity matters
The best data science organizations have incredible diversity in their datasets, as well as among their teams. These new CDOs can choose to work in isolation within a single agency or network and bring in those not only from other agencies, but also from industry and academia. Those that work more broadly will see more significant results.
CDOs should also approach hiring from this broad network. Talent in data science is not easy to find, but opening the door to the broader market will diversify problem solving and perspective and increase the potential to find the best talent. In fact, world-class organizations often bring people in from all of ethnic, gender and religious backgrounds and frequently have a high blend of disciplines and backgrounds. These different backgrounds and perspectives prove to be exceptionally valuable in data science, especially when working to solve incredibly complex problems. Diverse views can counterbalance biases that can influence outcomes, helping to create better analysis.
3. Create datasets with a problem in mind
Many organizations have transitioned from having CDOs to having chief data and analytics officers (CDAO). Why? The answer is simple: Today's data landscape is complicated by large collections of data that are poorly structured, documented and maintained -- often referred to as data swamps. These data repositories are not well curated and quickly lose value as an asset, and instead become sinkholes. To avoid the sinkhole syndrome, CDAOs should land data with an understanding of how it will be used or analyzed. It is now imperative that when structuring data, CDAOs remain focused on a specific problem to ensure they draw valuable insights.
4. Provide data as close to the grain as possible
There is an array of government datasets that are aggregated to the national, state or sometimes local levels. In most cases, the more granular the data, the more useful it becomes. While the work to anonymize data and to protect privacy goes up the deeper one goes, the value the more detailed data provides is frequently orders of magnitude improved.
5. Leverage existing best-in-breed technologies
With numerous examples of open data stores around the world, benchmarking and leveraging existing technology and tools will accelerate the journey. For example, monitoring tools allow data scientists to understand who is using data and how they are using it, search tools can help find specific data more easily, and fast data stores can easily download large datasets quickly.
One of the most useful tools CDOs can implement is community platforms that use existing, best-practice methods to provide ratings, commenting capabilities and contribution features. Kaggle Data, a community of online data scientists, is a great example of a platform CDOs can leverage to build models based on publicly available datasets.
6. Maintain culture
Engineering can be a difficult and thankless job, and the teams that are celebrated the most are often those that are on the front lines, building the solutions that the datasets inform. To truly cultivate a data-driven culture, CDOs must make sure their teams understand the value they create and stay connected through the process to see end results where possible.
One way to do this is to celebrate the team's success and create metrics around data use and economic value creation. Collaboration can also play a large role in this -- providing forums and venues for the data engineers to work side-by-side with those further down the value stream can help encourage junior data workers to grow into new roles and see the value of their work.
The soon-to-be-released Federal Data Strategy is brimming with potential to help drive new levels of analytics and faster solutions to some of our country's most challenging problems. Taking on the role of a new CDO for a government agency comes with its nuances, and to ensure success, emerging government CDOs need to be mindful of far more than simply structuring datasets.
NEXT STORY: Quick Hits