A Popular Algorithm Is No Better at Predicting Crimes Than Random People
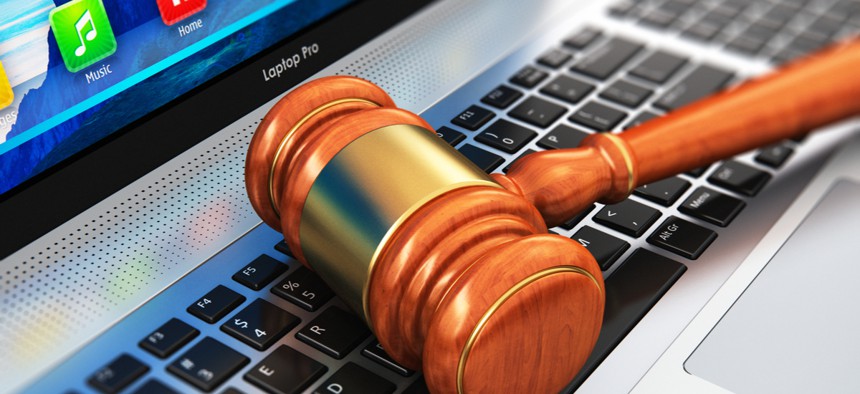
Scanrail1/Shutterstock.com
The COMPAS tool is widely used to assess a defendant’s risk of committing more crimes, but a new study puts its usefulness into perspective.
In February 2013, Eric Loomis was found driving a car that had been used in a shooting. He was arrested, and pleaded guilty to eluding an officer. In determining his sentence, a judge looked not just to his criminal record, but also to a score assigned by a tool called COMPAS.
Developed by a private company called Equivant (formerly Northpointe), COMPAS—or the Correctional Offender Management Profiling for Alternative Sanctions—purports to predict a defendant’s risk of committing another crime. It works through a proprietary algorithm that considers some of the answers to a 137-item questionnaire.
COMPAS is one of several such risk-assessment algorithms being used around the country to predict hot spots of violent crime, determine the types of supervision that inmates might need, or—as in Loomis’s case—provide information that might be useful in sentencing. COMPAS classified him as high-risk of re-offending, and Loomis was sentenced to six years.
He appealed the ruling on the grounds that the judge, in considering the outcome of an algorithm whose inner workings were secretive and could not be examined, violated due process. The appeal went up to the Wisconsin Supreme Court, who ruled against Loomis, noting that the sentence would have been the same had COMPAS never been consulted. Their ruling, however, urged caution and skepticism in the algorithm’s use.
Caution is indeed warranted, according to Julia Dressel and Hany Farid from Dartmouth College. In a new study, they have shown that COMPAS is no better at predicting an individual’s risk of recidivism than random volunteers recruited from the internet.
“Imagine you’re a judge and your court has purchased this software; the people behind it say they have big data and algorithms, and their software says the defendant is high-risk,” says Farid. “Now imagine I said: Hey, I asked 20 random people online if this person will recidivate and they said yes. How would you weight those two pieces of data? I bet you’d weight them differently. But what we’ve shown should give the courts some pause.” (A spokesperson from Equivant declined a request for an interview.)
COMPAS has attracted controversy before. In 2016, the technology reporter Julia Angwin and colleagues at ProPublica analyzed COMPAS assessments for more than 7,000 arrestees in Broward County, Florida, and published an investigationclaiming that the algorithm was biased against African Americans. The problems, they said, lay in the algorithm’s mistakes. “Blacks are almost twice as likely as whites to be labeled a higher risk but not actually re-offend,” the team wrote. And COMPAS “makes the opposite mistake among whites: They are much more likely than blacks to be labeled lower-risk but go on to commit other crimes.”
Northpointe questioned ProPublica’s analysis, as did various academics. They noted, among other rebuttals, that the program correctly predicted recidivism in both white and black defendants at similar rates. For any given score on COMPAS’s 10-point scale, white and black people are just as likely to re-offend as each other. Others have noted that this debate hinges on one’s definition of fairness, and that it’s mathematically impossible to satisfy the standards set by both Northpointe and ProPublica—a story at The Washington Post clearly explains why.
The debate continues, but when Dressel read about it, she realized that it masked a different problem. “There was this underlying assumption in the conversation that the algorithm’s predictions were inherently better than human ones,” she says, “but I couldn’t find any research proving that.” So she and Farid did their own.
They recruited 400 volunteers through a crowdsourcing site. Each person saw short descriptions of defendants from ProPublica’s investigation, highlighting seven pieces of information. Based on that, they had to guess if the defendant would commit another crime within two years.
On average, they got the right answer 63 percent of their time, and the group’s accuracy rose to 67 percent if their answers were pooled. COMPAS, by contrast, has an accuracy of 65 percent. It’s barely better than individual guessers, and no better than a crowd. “These are nonexperts, responding to an online survey with a fraction of the amount of information that the software has,” says Farid. “So what exactly is software like COMPAS doing?”
Only Equivant can say, and they’re not revealing the secrets of their algorithm. So the duo developed their own algorithm, and made it as simple as possible—“the kind of thing you teach undergrads in a machine-learning course,” says Farid. They found that this training-wheels algorithm could perform just as well as COMPAS, with an accuracy of 67 percent, even when using just two pieces of data—a defendant’s age, and their number of previous convictions. “If you are young and have a lot of prior convictions, you are high-risk,” says Farid. “It’s kind of obvious.”
Other teams have found similar results. Last year, Cynthia Rudin, from Duke University, showed that a basic set of rules based on a person’s age, sex, and prior convictions—essentially, an algorithm so simple you could write it on a business card—could predict recidivism as well as COMPAS.
The problem isn’t necessarily that COMPAS is unsophisticated, says Farid, but that it has hit a ceiling in sophistication. When he and Dressel designed more complicated algorithms, they never improved on the bare-bones version that used just age and prior convictions. “It suggests not that the algorithms aren’t sophisticated enough, but that there’s no signal,” he says. Maybe this is just as good as it gets. Maybe the whole concept of predicting recidivism is going to stall at odds that are not that much better than a coin toss.
Sharad Goel, from Stanford University, sees it a little differently. He notes that judges in the real world have access to far more information than the volunteers in Dressel and Farid’s study, including witness testimonies, statements from attorneys, and more. Paradoxically, that informational overload can lead to worse results by allowing human biases to kick in. Simple sets of rules can often lead to better risk assessments—something that Goel found in his own work. Hence the reasonable accuracy of Dressel and Farid’s volunteers, based on just seven pieces of information.
“That finding should not be interpreted as meaning that risk-assessment tools add no value,” says Goel. Instead, the message is “when you tell people to focus on the right things, even nonexperts can compete with machine-learning algorithms.”
Equivant make a similar point in a response to Dressel and Farid’s study, published on Wednesday. “The findings of ‘virtually equal predictive accuracy’ in this study,” the statement says, “instead of being a criticism of the COMPAS assessment, actually adds to a growing number of independent studies that have confirmed that COMPAS achieves good predictability and matches the increasingly accepted AUC standard of 0.70 for well-designed risk assessment tools used in criminal justice.”
There have been several studies showing that algorithms can be used to positive effect in the criminal-justice system. “We’re not saying you shouldn’t use them,” says Farid. “We’re saying you should understand them. You shouldn’t need people like us to say: This doesn’t work. You should have to prove that something works before hinging people’s lives on it.”
“Before we even get to fairness, we need to make sure that these tools are accurate to begin with,” adds Dressel. “If not, then they’re not fair to anyone.”