Machine-Learning Technologies Help Agencies Develop Highly Intelligent Security Postures
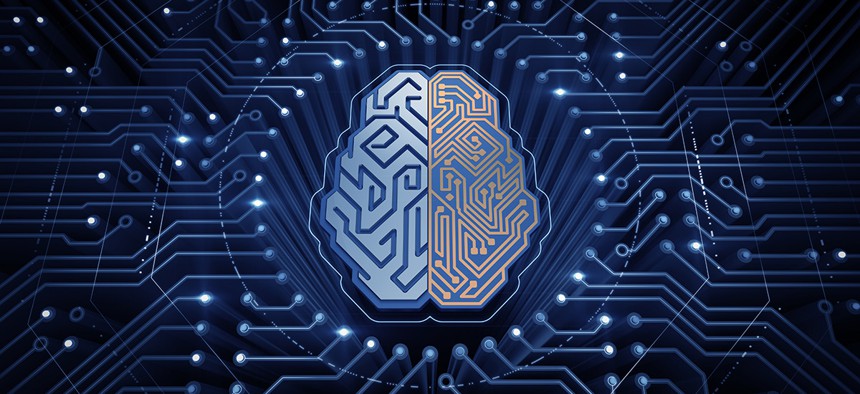
Sergey Tarasov/Shutterstock.com
Machine learning makes life much more manageable for network security operators.
Dave Mihelcic is the head of federal strategy and technology at Juniper Networks.
If the recent spate of alleged Russian cyberattacks has taught us anything, security breaches can happen so quickly and stealthily, the damage will be done before anyone even realizes there was a hack.
In fact, as malicious actors become more insidious, federal network security managers are finding the reaction time between identifying and mitigating potential threats has gone from minutes to milliseconds. Factor in the volume and complexity of the threats, and it becomes evident the challenge has grown well beyond what can be managed through manual intervention.
» Get the best federal technology news and ideas delivered right to your inbox. Sign up here.
To successfully combat these challenges, cyber operators should consider incorporating machine-learning capabilities into their toolkit. Once used within the Defense Department primarily for real-world target recognition, machine-learning technologies have evolved to become very effective at quickly detecting and responding to potential cyber threats. Through analytics and predetermined risk factors established by cyber operators, these highly intelligent and adaptable systems can evolve to “learn” about threats as they happen and apply that knowledge to better fortify the network in anticipation of future threats.
Machine-learning tools can interact with other components of the network infrastructure to create a remarkable level of advanced threat protection. The tools can continuously evaluate and monitor web and email files in the hunt for evasive malware and use various cloud-based technologies and resources to identify risks.
They can also be used in combination with other network security solutions, including firewalls and edge and core routing and switching infrastructures, to fend off attacks and isolate infected hosts.
Let’s take a look at a hypothetical example to illustrate how machine learning works for cybersecurity. An agency’s analytics-based machine learning system may include a predetermined set of risk factors. When the system has detected enough of these risk factors have been triggered, it will take a predetermined action to help protect the network—for example, blocking access to the network.
At this point, the network security operator can step in and help “teach” the machine. If the operator examines the incident and determines it does not pose a threat, the IT team may remove some of the mitigation protocols. This effectively trains the machine to recognize something was not a hostile attack, and it is OK to ignore this type of event in the future.
Or the operator can confirm the machine’s action by allowing the block to continue. This effectively confirms to the machine an attack is underway and alerts it that it should respond accordingly to similar events in the future. Over time, the system becomes trained to intelligently determine whether or not the risk factors it is detecting indicate a hostile cyberattack.
It should be noted the treasure trove of real-time network monitoring data and analytics federal organizations have at their disposal can be an effective cybersecurity resource when used in conjunction with machine-learning tools. Instead of having predetermined analytics that always comes up with the same answers to the same questions, analytics can be adjusted and evolve over time to better respond to potential risks.
Machine learning can have a positive impact beyond enhanced security and decreased risk of hostile attacks because it can be used to create a more efficient and automated security apparatus that reduces operator workloads. The combination of machine learning with other automated network technologies, such as software-defined networking and cloud solutions, can allow operators to do more with less and free up time to pursue other mission-critical activities.
It also minimizes the risk of human error and lays the groundwork for faster development of more robust and complex systems that can effectively combat threats with minimal human intervention.
At some point, we must acknowledge without massive automation, there simply are not enough humans on Earth to manage IT infrastructures and security operations globally. Network technologies like SDN scale far too quickly, and security threats are too advanced, to leave the management of these solutions solely in the hands of human beings.
Therefore, we have no choice but to make network infrastructures more programmable, autonomous and secure. Machine learning checks off all of these boxes, all while making life much more manageable for network security operators.