A Computer’s Hot Take on the 2016 Election
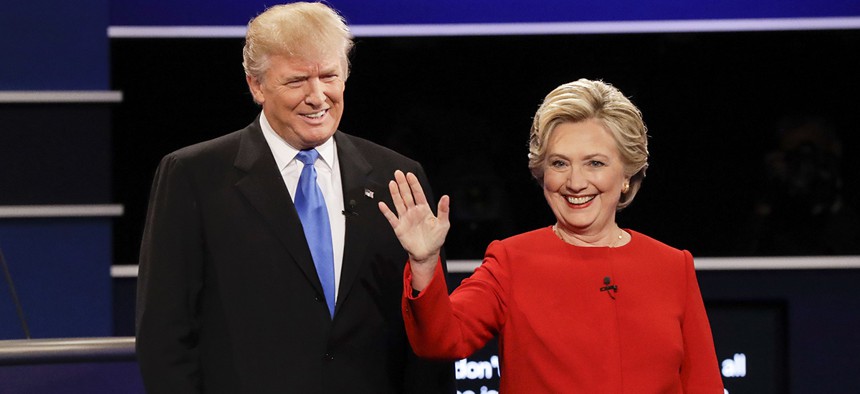
Republican presidential nominee Donald Trump and Democratic presidential nominee Hillary Clinton are introduced during the presidential debate at Hofstra University in Hempstead, N.Y., Monday, Sept. 26, 2016. David Goldman/AP File Photo
An artificial intelligence found Bernie Sanders and Hillary Clinton seemed to get “happier” coverage than Donald Trump. But is that evidence of media bias? Not necessarily.
Donald Trump’s message of the week, in case you’ve somehow managed to avoid it, is that the election is rigged by a corrupt (and apparently monolithic) media.
“The election is absolutely being rigged by the dishonest and distorted media pushing Crooked Hillary,” Trump wrote in a tweet on Sunday night.
Trump has said repeatedly he’s been treated unfairly by news organizations. But is that true?
Back in July, I asked the computer scientist Andy Reagan if he could help me design an experiment that might begin to gauge the tone of media coverage about various presidential candidates. I knew Reagan, who is working toward his Ph.D. in mathematics at the University of Vermont, from his fascinating research into how a computer identified the six main arcs in storytelling across literary fiction. For that work, he and his colleagues had developed a database of more than 10,000 frequently-used words, all ranked by how “happy” they were perceived to be. (The happiest word on their list: Laughter. The saddest: Terrorist.)
I wondered if it would be possible to use that list—and Reagan’s computer skills—to assess a huge trove of campaign coverage over time, so we could compare the overall tone of articles about the different candidates. But to do this, we would need a ginormous database of campaign coverage.
Digging into the Nexis newspaper archives, I collected tens of thousands of articles about Bernie Sanders, Hillary Clinton and Donald Trump—whatever was published in more than 50 U.S. newspapers and websites over a 13-month period from July 2015 through August 2016. (I also pulled a year’s worth of campaign coverage about Bill Clinton from his 1992 run for president; more on that in a minute.)
Reagan then took the data and fed it into his computer model, which spit out a complex portrait of the tone of campaign coverage over the past year. We had the beginnings of a sentiment analysis of presidential campaign coverage, one that might suggest the emotional tone of media stories about each candidate.
Trump may feel validated to know that, indeed, we found that articles about him, overall, were the most negative of the bunch—even before the utter insanity of the past several weeks. But there are plenty of caveats.
First, let’s look at the data. A totally neutral article—one with just as many happy words as sad words—would have scored a 5.0 on Reagan’s ranking system. Sanders, Clinton and Trump all scored higher than that—meaning, the collection of articles about each of them were more positive than negative. That’s not surprising, Reagan told me, because natural language is biased toward happy words.
The 18,640 articles about Hillary Clinton that we assessed scored 5.85 on the happiness scale, whereas the 29,019 stories about Trump from that same period ranked 5.78—not quite as happy. Bernie Sanders scored the highest for the positive tone of coverage about him, with a ranking of 5.90 across 7,841 articles. (For context on what these numbers mean, consider the happiest day on Twitter in the past year: Christmas day, according to Reagan’s model, with a score of 6.28.)
It isn’t clear from the number-rankings alone, but the differences we found in coverage are meaningful—if not exactly dramatic. The scores themselves are “only useful in relative terms,” Reagan told me. And they’re most interesting once you dig into the substance of why coverage about one candidate is more positive or negative than the next.
On a word-by-word basis, we can see why coverage of Trump skews more negative—and in many cases, it seems to be because the coverage about him reflects the language he himself uses.
So, for instance, the word “great” appears frequently in articles Trump, whose campaign slogan is “Make America Great Again.” And because “great” is perceived as an exceedingly positive word—it ranks 55th happiest on a list of more than 10,000 words—this helps buoy his score. But there are many other negative words frequently associated with Trump—more so than with Hillary Clinton—that drag it back down, including: “bad,” “racist,” “war,” “no,” “don’t,” “ban” and “illegal.”
This doesn't come as much of a surprise: Some of Trump’s loudest campaign messages have been about his call for a ban on Muslims entering the United States—or, in his words a “complete and total shutdown”—and his belief that illegal immigrants “have to go.”
Tonally, many observers noted a deep contrast in rhetoric between Trump and Clinton at the conventions in July—Trump’s speech was widely perceived as far more pessimistic than Clinton's. Put another way, a sentiment analysis of the candidates’ words—just verbatim transcripts, with no added reporting—might still find a more negative ranking for Trump.
Looking at the words that influence the sentiment score for articles about Hillary Clinton, we find words with positive associations like: “new,” “like,” “win,” “first,” “you,” “united” and “women.” And despite the negative words associated with coverage of the Democratic candidate (“gun,” “attacks,” “fight,” “tax,” “war,” “never,”) the overall picture is more positive for Clinton than it is for Trump.
Coverage of Bernie Sanders ranked “happiest” of all the candidates—and, interestingly, his score was defined by a notably higher prevalence of positive words than his political peers. Once again, as with Trump, you can catch a glimpse of Sanders’s campaign rhetoric in the articles about him—articles that were characterized by only a handful of negative words (“poverty,” and “fight” were among the few) but many positive ones (“win,” “health,” “new,” “victory,” “free,” “young” and “college.”)
There’s a pretty significant contextual problem to taking overall scores at face value, though.
For one thing, there are two words in particular that are weighted as positive, but that represent decidedly negative elements of coverage of the 2016 campaign: “Email” and “women.”
So, while the computer model would tell us that more mentions of “email” would give coverage of any candidate a higher score on the happiness scale—more mentions of “email” is, in actuality, a potential negative for Clinton, given her improper use of a private email account during her time as secretary of state.
Similarly, up until August, there were more mentions of “women” in articles about Clinton than there were in articles about Trump—a finding that contributed to Trump’s lower score on the happiness scale. But the many mentions of “women” in stories about Trump since then, given the repeated accusations of sexual assault he is facing, are decidedly negative in context, despite how a computer model would see it.
There’s a similar contextual absence in the computer’s assessment of tone among stories about Bill Clinton, in 1992. One of the happiest words that appears frequently in stories about him, and a word that helps inflate his overall score, is “flowers.”
But anyone who remembers the 1992 campaign is likely to recognize that the many references to “flowers” were probably references to “Gennifer Flowers,” the woman who came forward during Bill Clinton’s run for presidency and said she’d had an affair with him. Not so positive, after all.
“There are always going to be words that are used in different contexts, and sometimes the difference is subtle,” Reagan told me. This is exactly the reason it’s important to use methods where a computer’s model can be inspected on a word-by-word basis after the fact, he says, rather than a deep learning approach that would be impossible to reverse engineer.
Of course, there’s a larger point still: Journalistic fairness doesn’t come down to how happy each word in the article makes a reader feel—even when the word is weighted properly in context of the events it’s describing. Nor does every candidate merit the same kind of coverage. Often, the tone of a story is negative because it reflects the reality of what’s happening. And no one should need a computer model to recognize that.