Why Big Data Needs ‘Dummy Data’
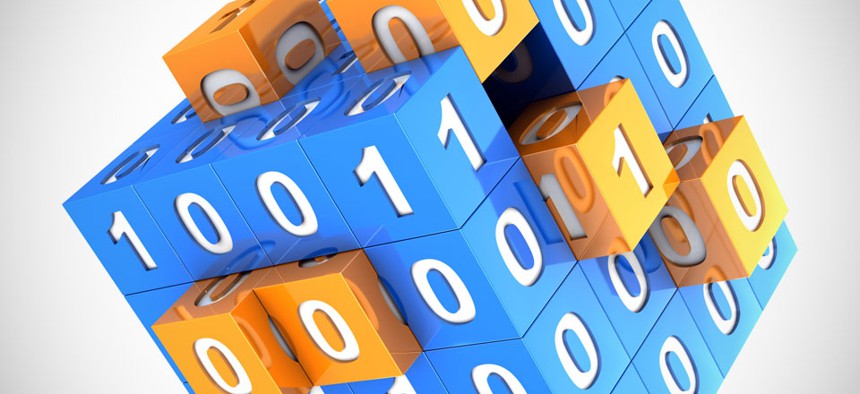
Mmaxer/Shutterstock.com
Instead of original data, a structurally similar but obscured version of the data, or “dummy data”, is used for development and testing environments.
Ted Girard is a vice president at Delphix Federal.
The amount of data the U.S. produces has exploded in recent years; it is central to practically everything we do.
There is no question big data is, in fact, a big deal. In 2012, the Obama administration announced a $200 million commitment by six agencies to invest in big data projects.
Big data can enable important discoveries and innovations in public safety, health care and medicine, education, national defense and many other areas. It also has the potential to save nearly $500 billion – or 14 percent of the total spent on agency budgets – across the federal government.
The success of federal missions largely depends on the timeliness and quality of data. Yet, the exponential growth in data is making it difficult to distribute the right information to constituents when they need it. This big data emphasis requires new tools and technologies to help agencies use this data for their strategic advantage.
While the big data revolution presents immense opportunities, there are also profound implications and new challenges associated with it, including how best to protect privacy, enhance security and improve data quality. For many agencies just getting started with their big data efforts, these challenges can prove overwhelming.
Why Data Masking?
The protection of sensitive health, educational and financial information is so critical, numerous privacy regulations exist that require test data sets to be protected.
Data masking – a technique used to ensure sensitive data does not enter nonproduction systems – is designed to protect the original production data from individuals or project teams that do not need real data to perform their tasks.
Instead of original data, a structurally similar but obscured version of the data, or “dummy data”, is used for development and testing environments.
The problem with traditional data masking solutions is they are static – this means that every request by users for new or refreshed data sets must go through the manual masking process each time. This is a cumbersome and time-consuming process that promotes “cutting corners” – skipping the process altogether and using old, previously masked data sets or delivering teams unmasked versions.
To meet the new demands for security and speed of data delivery, new agile data masking solutions have been developed. Agile data masking combines the processes of masking and provisioning, allowing organizations to quickly and securely deliver protected data sets in minutes.
To gain the most advantage from big data, agencies should understand three key data attributes – security and privacy, quality and agility.
Data Security and Privacy
While not all government agencies deal with sensitive data related to defense or national security, most agencies do collect, store and process personal, financial, health and other information that must be protected. Information security and privacy considerations are daunting challenges for federal agencies and may be hindering their efforts to pursue big data programs.
The good news is, advanced agile masking technology has emerged to help agencies raise the level of security and privacy assurance and meet regulatory compliance requirements. Agile masking enables developers to work with fresh data without risking a compromise – sensitive data is protected at each step in the life cycle automatically.
Data Quality
Some IT experts argue the greatest impact of big data is on data quality.
Poor data is, in fact, a leading cause of many IT project failures. Creating better, faster and more robust means of accessing and analyzing large data sets is imperative to keep pace. Preserving value and maintaining integrity while protecting data privacy is extremely difficult.
As data migrates across systems, controls need to be in place to ensure no data is lost, corrupted or duplicated in the process. The key to effective data masking is making the process seamless to the user so that new data sets are complete and protected while remaining in sync across systems.
Traditionally, the cumbersome process of masking data has led users to compromise data quality by using stale, artificial or limited data sets. Implementing agile data masking solutions enables organizations to be assured that test and development tasks will be performed with the right data sets in a secure manner.
Agile Data Masking
Security and privacy do not have to come at the cost of speed and quality. Big data projects will continue to be among the top priorities for government agencies. Agencies need to be able to quickly and securely analyze vast amounts of data in real time in order to make faster, more informed decisions.
For this to occur, agencies need to create an agile data management environment to process, analyze and manage data and information. Agile data masking will help kick start or transform agency big data initiatives by unlocking data to achieve agency missions.
(Image via Mmaxer/Shutterstock.com)